Introducing V-JEPA: Teaching Machines to Understand the Physical World Through Videos
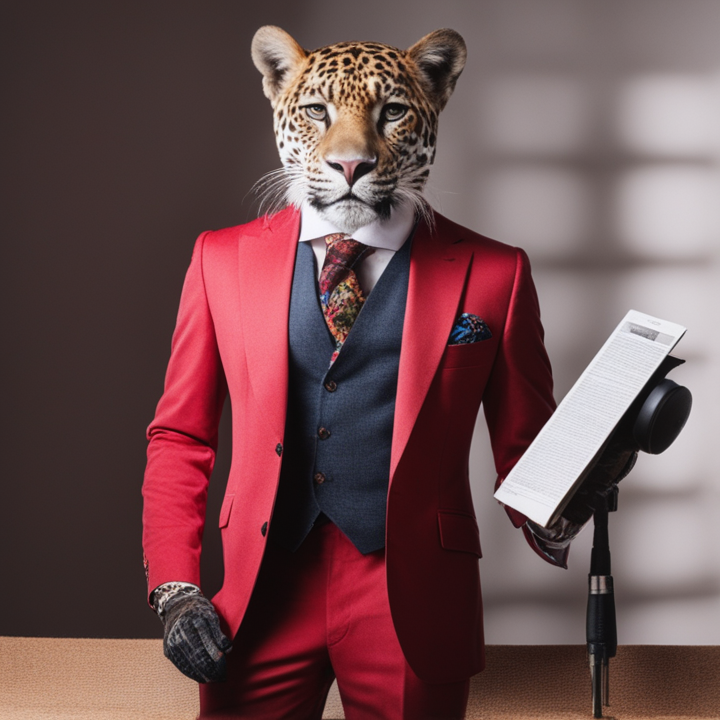
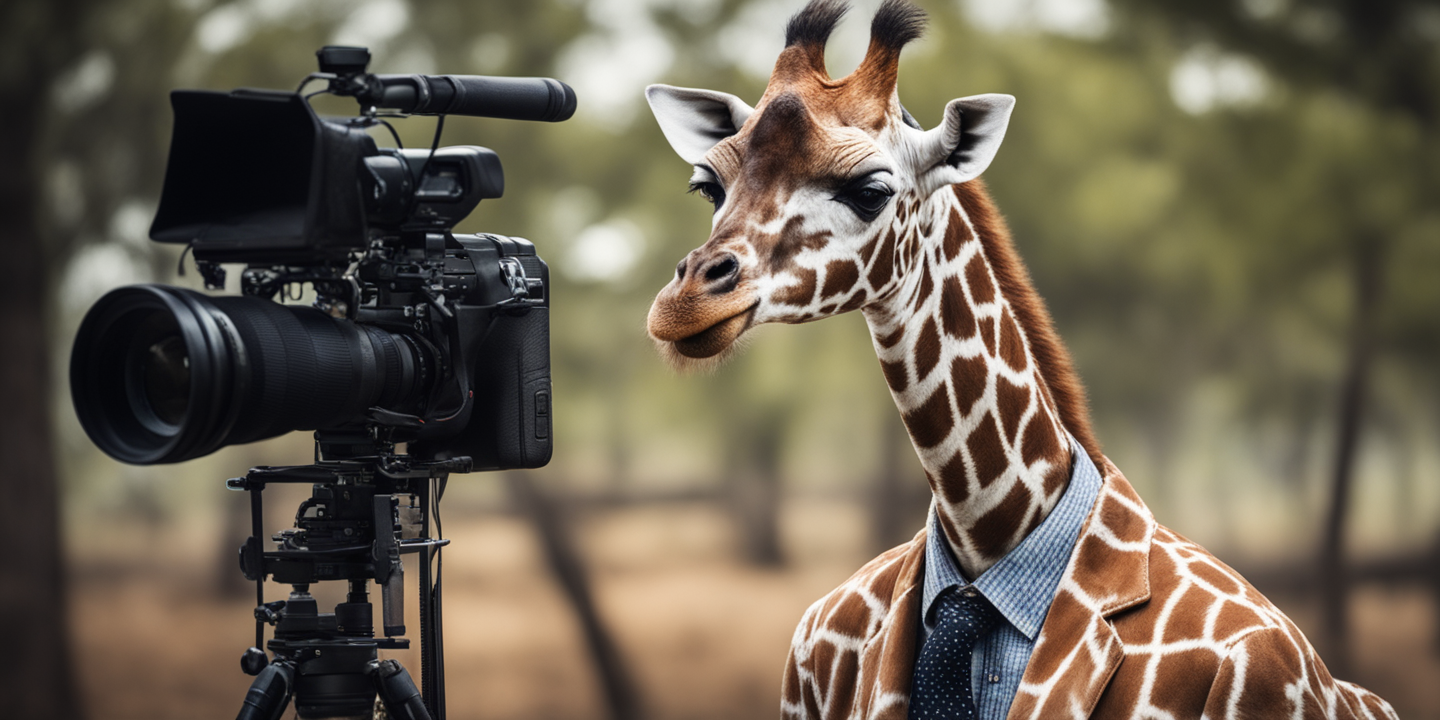
Discover V-JEPA, a groundbreaking method for imparting machines with the ability to comprehend and model the physical realm through video observation. This advancement, aligning with Yann LeCun’s AI vision, empowers models to plan, reason, and execute complex tasks by assimilating a learned understanding of the world. Explore the details and access the code via the provided links. As part of this release, delve into a suite of V-JEPA vision models, trained using self-supervised learning with a feature prediction objective. These models possess the capability to discern and forecast activities within videos, even with limited visual cues. Unlike traditional generative approaches, V-JEPA's methodology focuses on predicting missing or obscured segments within a video's abstract representation space. This flexible technique yields up to 6x enhancements in training and sample efficiency. The results demonstrate the competitiveness and, in some cases, superiority of our top V-JEPA models across benchmark datasets like Kinetics-400, Something-Something-v2, and ImageNet1K when employing a frozen backbone. This work signifies a significant stride towards advancing machine intelligence. Upholding our commitment to responsible open science, V-JEPA is released under a CC-BY-NC license, fostering collaboration and innovation within the research community.