RLOO: The New RLHF Champion
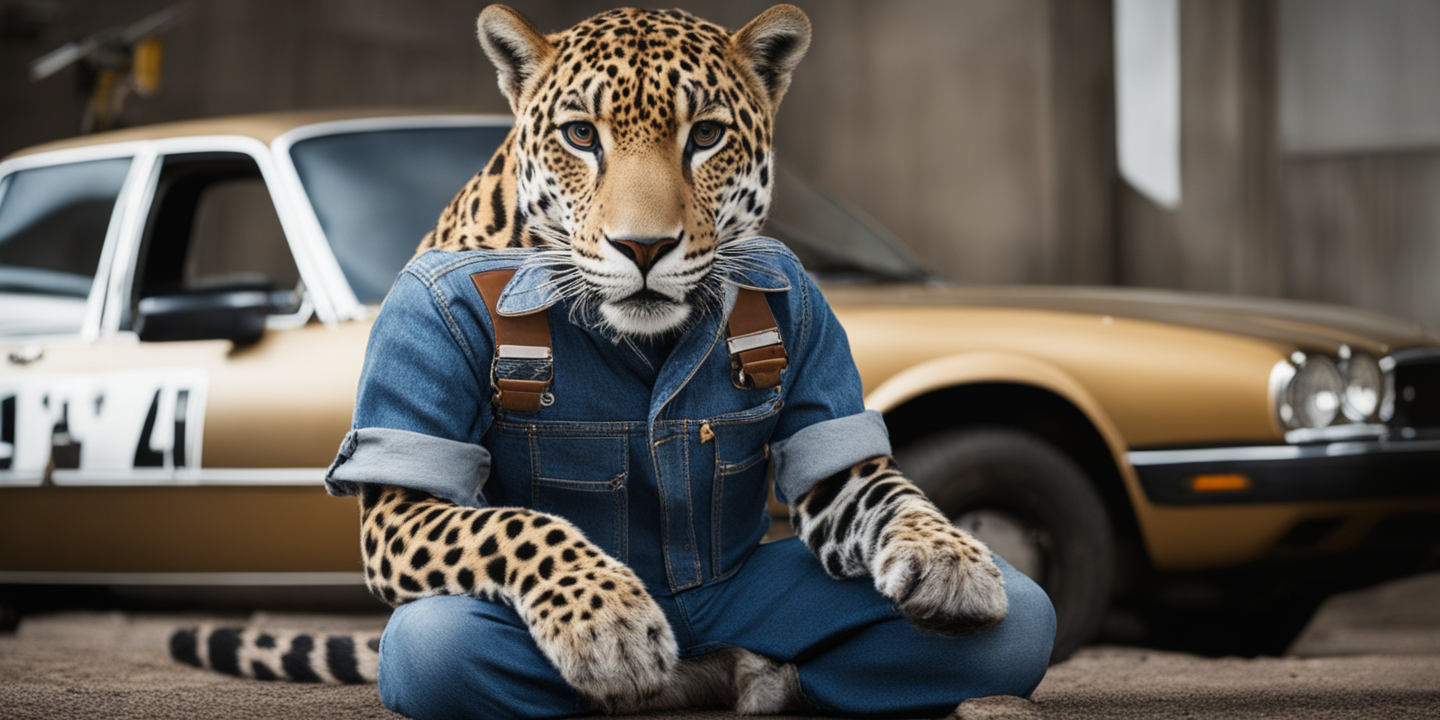
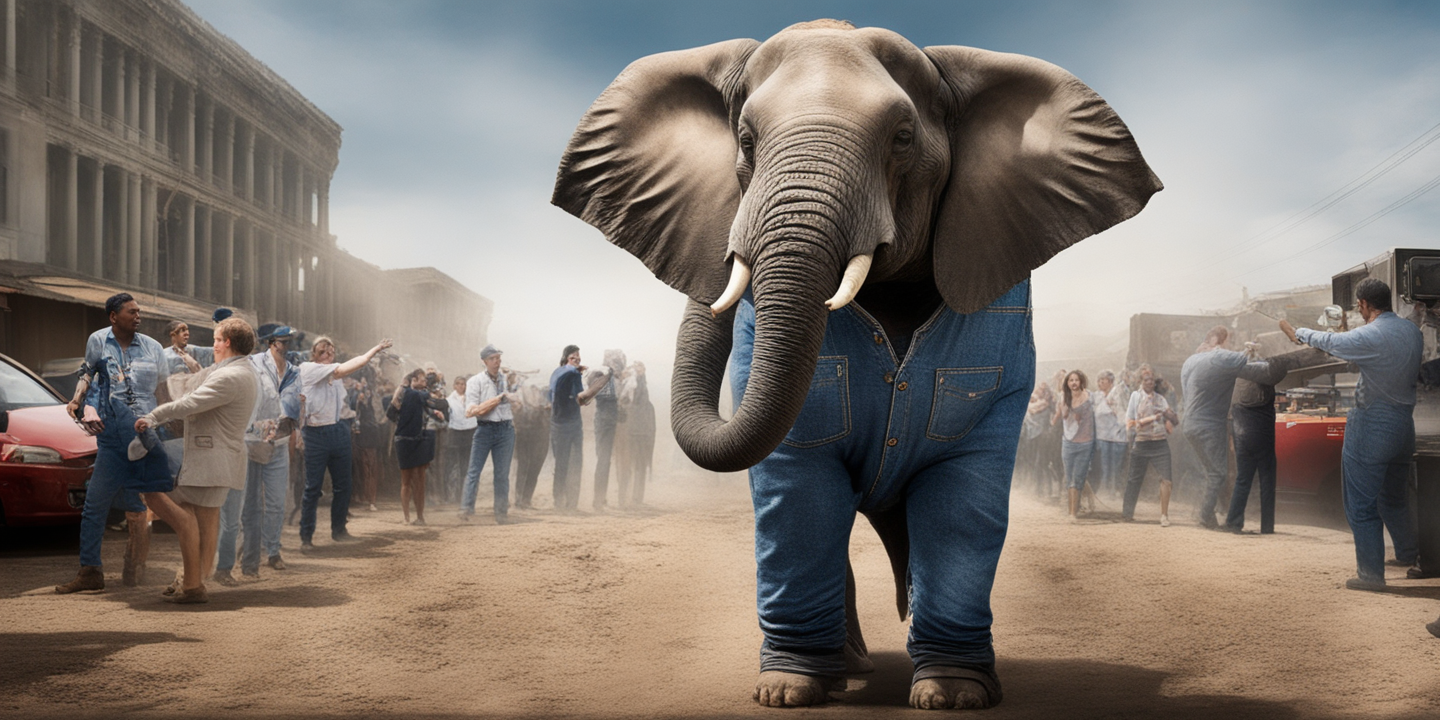
RLOO (Reinforcement Learning Leave-One-Out) is revolutionizing the RLHF (Reinforcement Learning from Human Feedback) training process by treating model completion as a single action and employing the REINFORCE algorithm. This method bypasses the need for a value model, thereby reducing memory requirements and enabling larger batch sizes. RLOO enhances REINFORCE with a leave-one-out mechanism that generates multiple samples per prompt and calculates rewards by averaging the other samples, minimizing gradient variance. RLOO requires 50-70% less memory and runs 2-3 times faster than PPO, needing only three model copies in memory. It also proves less sensitive to hyperparameter tuning. Achieving a 62.2% win rate on the Anthropic-HH dataset, RLOO outperforms RAFT, PPO, and DPO, with higher k values leading to even better results. This method is now available in Hugging Face TRL.