RLHF vs DPO: Navigating LLM Evolution
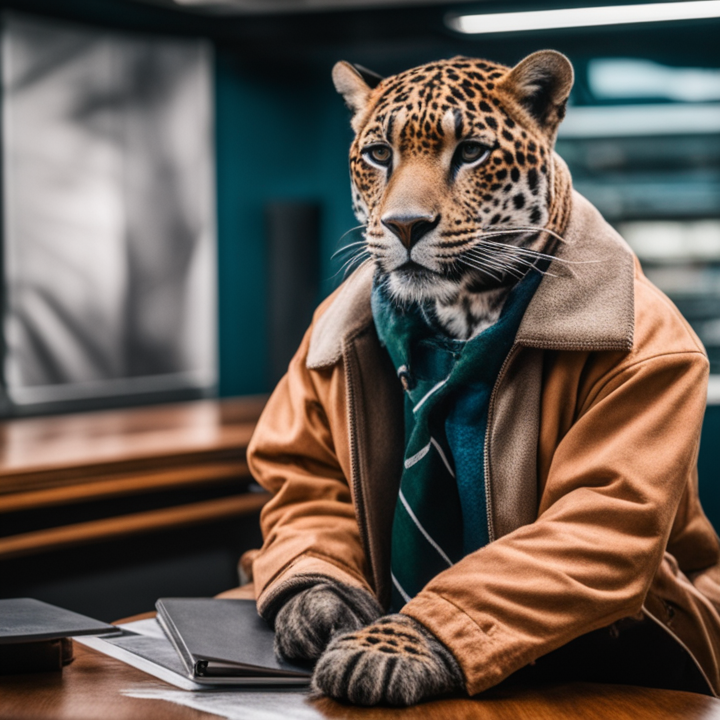
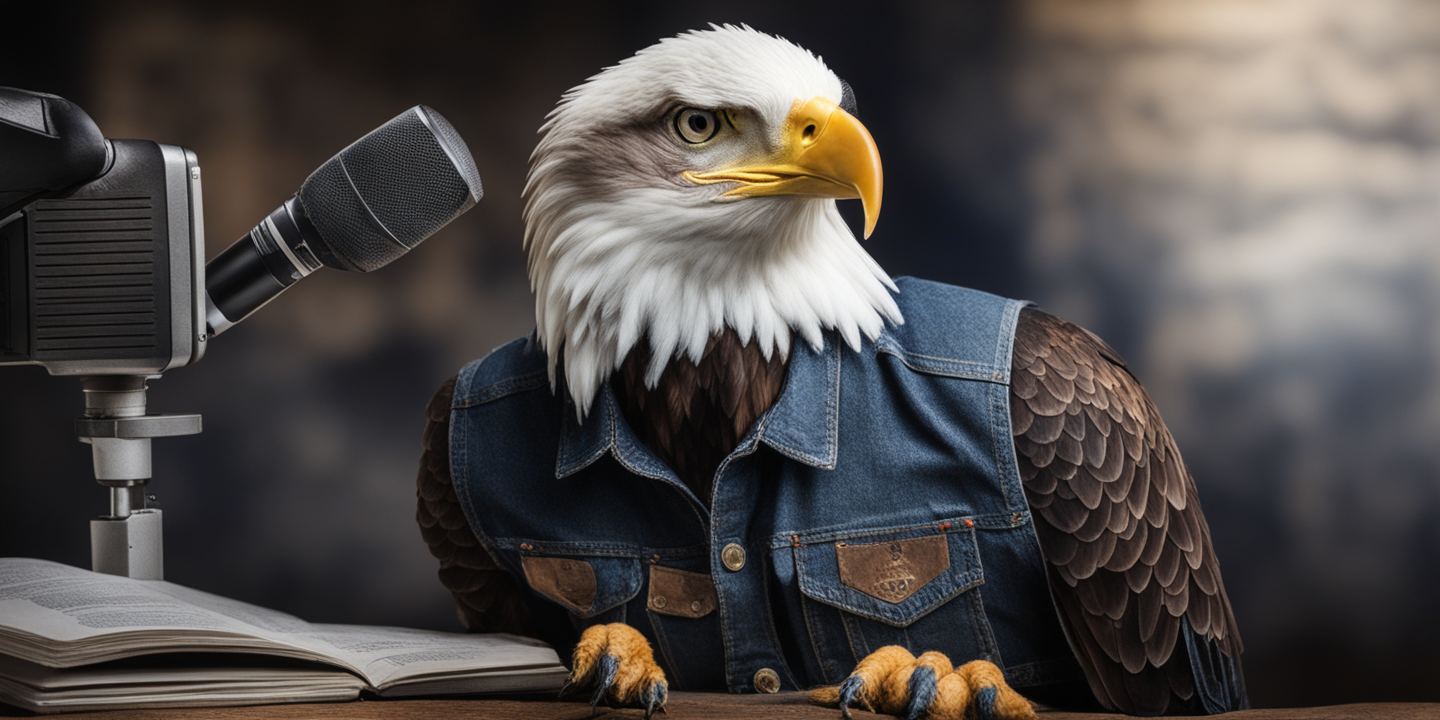
As large language models (LLMs) reshape human-machine interactions, the quest to refine their capabilities intensifies. Within this landscape, Direct Preference Optimization (DPO) and Reinforcement Learning from Human Feedback (RLHF) emerge as frontrunners, each offering distinct pathways to tailor LLMs to human needs. While DPO focuses on direct optimization based on predefined preferences, RLHF harnesses human feedback to guide model evolution. This article explores the nuances of both methodologies, shedding light on their respective strengths and applications in bridging the gap between LLM capabilities and human expectations.