RLHF's DPO Breakthrough
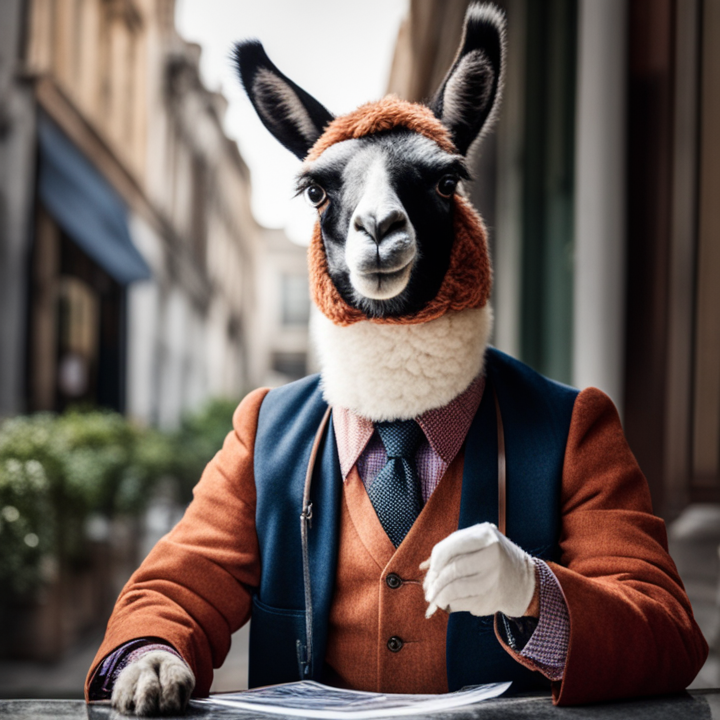
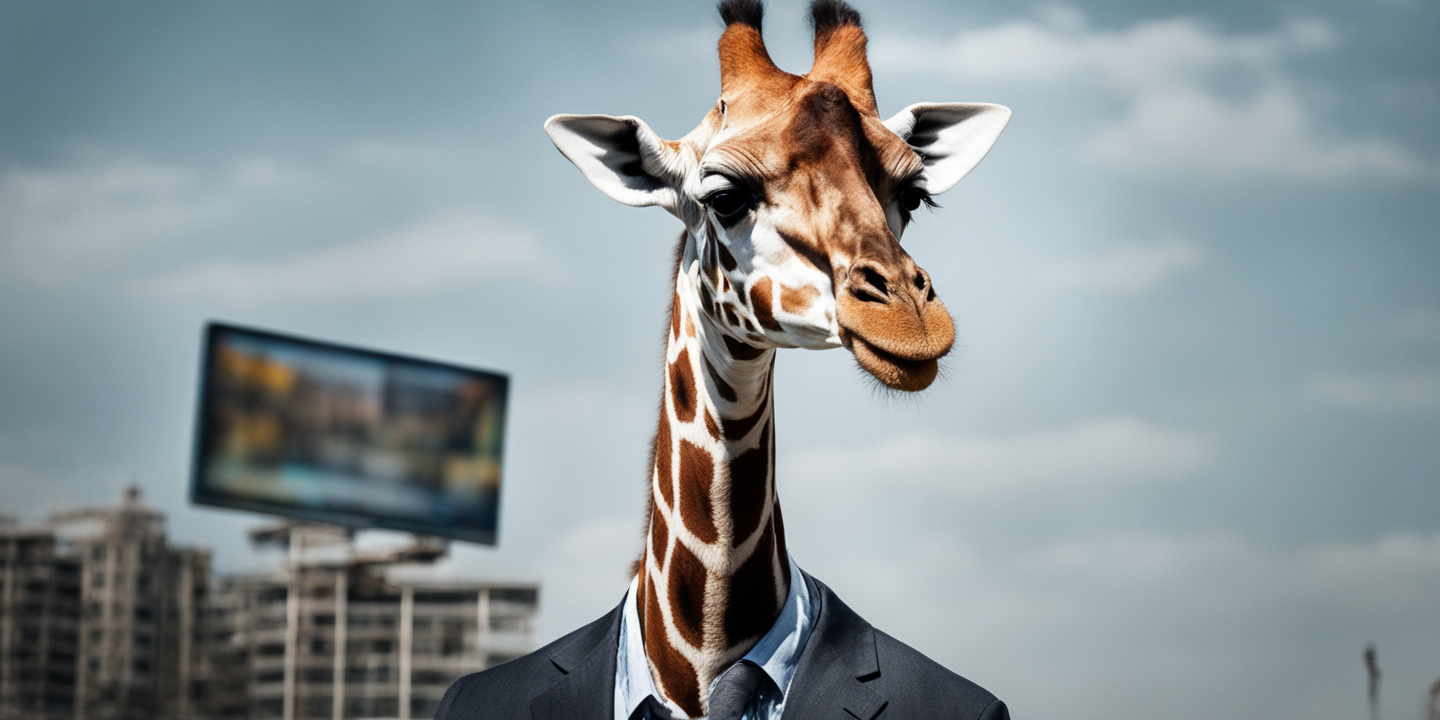
RLHF introduces a potential game-changer in preference tuning for Large Language Models (LLMs) with the advent of Direct Preference Optimization (DPO). Departing from the complexity of Reinforcement Learning from Human Feedback (RLHF), DPO offers a streamlined method that directly incorporates human input. As detailed in "Direct Preference Optimization: Your Language Model is Secretly a Reward Model," DPO emerges as a simplified yet potent alternative. While RLHF has set the standard for aligning AI outputs with human expectations, the emergence of DPO poses an intriguing question: Can it offer both increased efficiency and effectiveness in tuning models to human preferences? This article delves into the mechanics of DPO, highlights its distinctions from RLHF, and explores whether DPO represents the optimal choice for preference tuning in LLMs.