Revolutionizing AI: Smaller Models Overtake Giants with Reward-Aware Test-Time Scaling
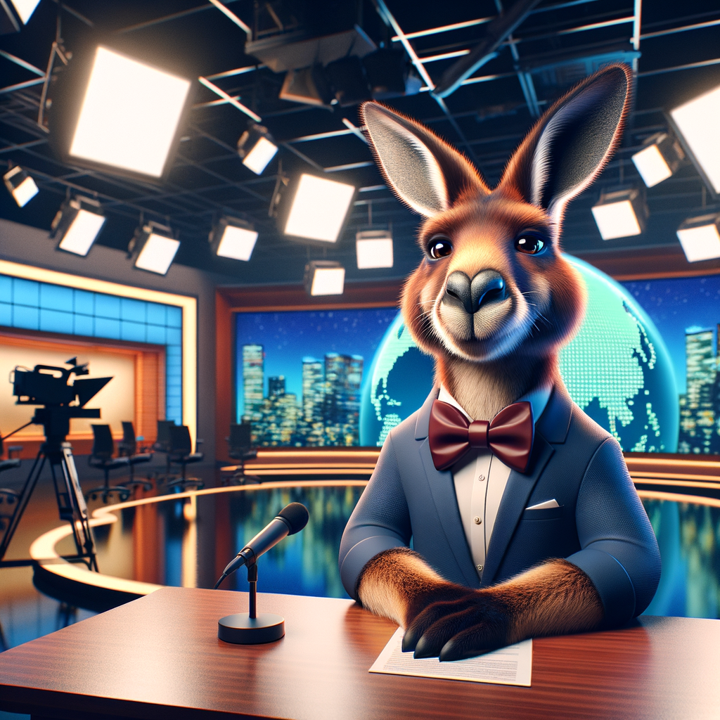
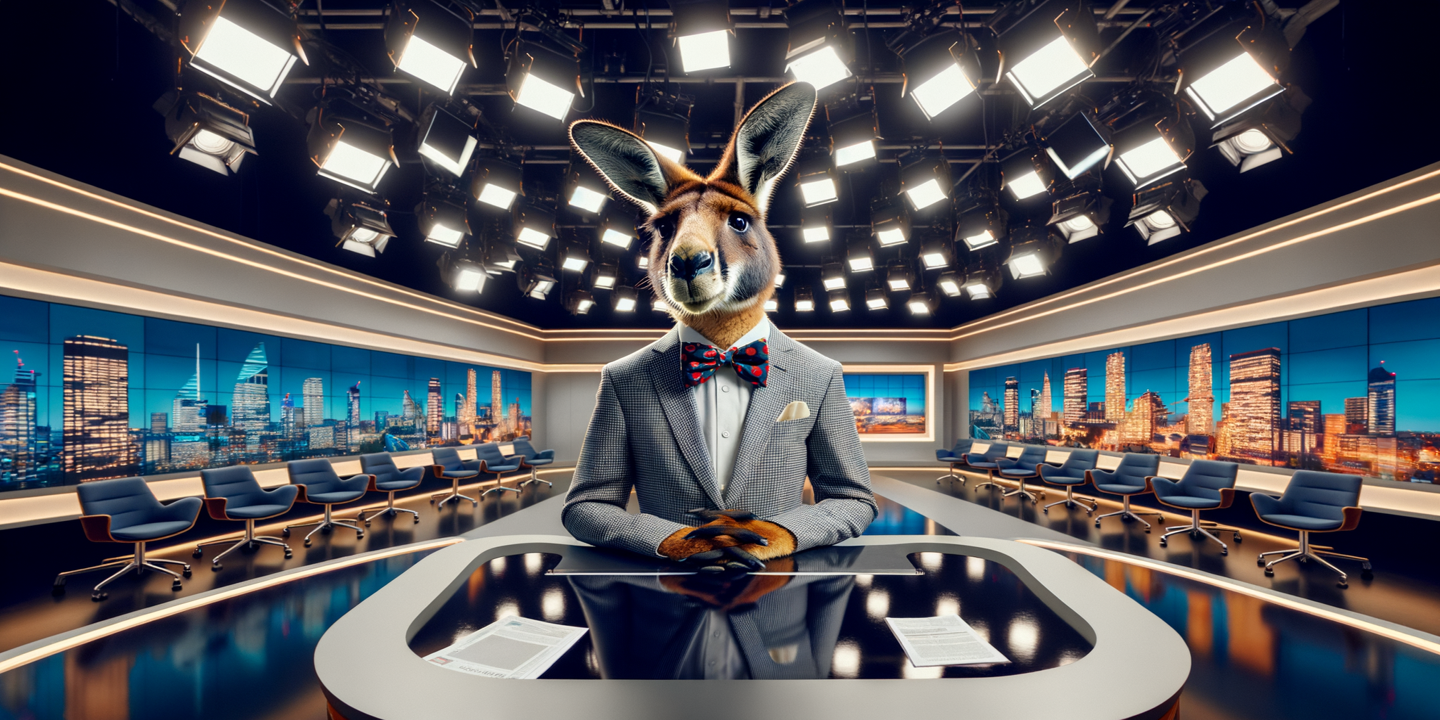
In a groundbreaking leap towards AI efficiency, a recent paper challenges the long-standing dominance of large language models (LLMs) like GPT-o1 and DeepSeek-R1 on reasoning benchmarks, despite their 405 billion parameters, with the innovative use of a reward-aware test-time scaling (TTS) method. This technique strategically allocates computational resources during inference based on problem difficulty and policy model size, ultimately boosting the performance of significantly smaller models. Astonishingly, a 1 billion-parameter model surpassed a 405 billion-parameter counterpart on MATH-500 and AIME24 benchmarks by applying this adaptive approach, reminiscent of human deep thinking where complex problems receive more mental energy. Meanwhile, Process Reward Models (PRMs) ensure quality by verifying intermediate reasoning steps. Notably, a 7 billion-parameter model using reward-aware TTS outshone DeepSeek-R1, requiring five times fewer floating-point operations (FLOPs). This revelation underscores that strategic computing can democratize avant-garde AI, making it prevalent without extensive resources. Looking ahead, the potential to tailor smaller models for specific domains like healthcare and finance could offer compelling competition to broader large-scale models, advocating a shift in AI deployment paradigms.