PatchTST and PatchTSMixer Join Transformers
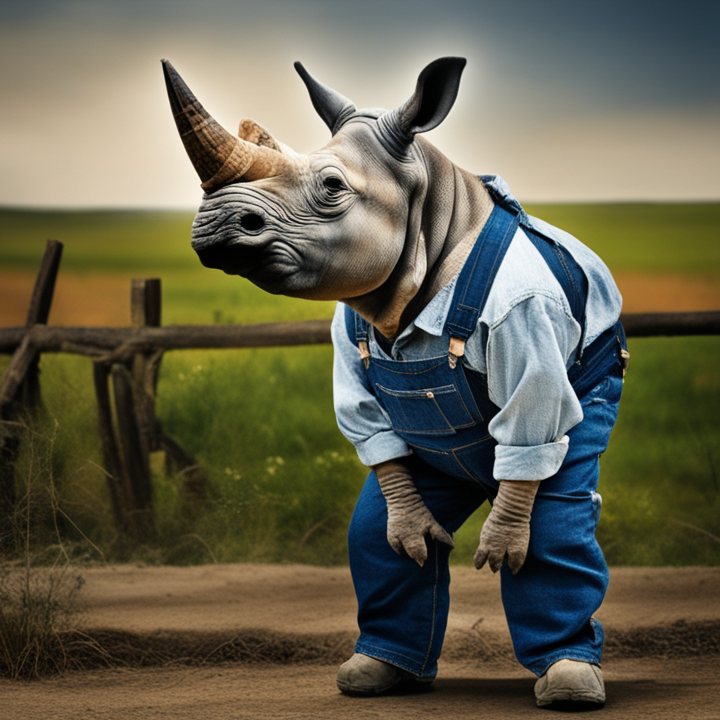
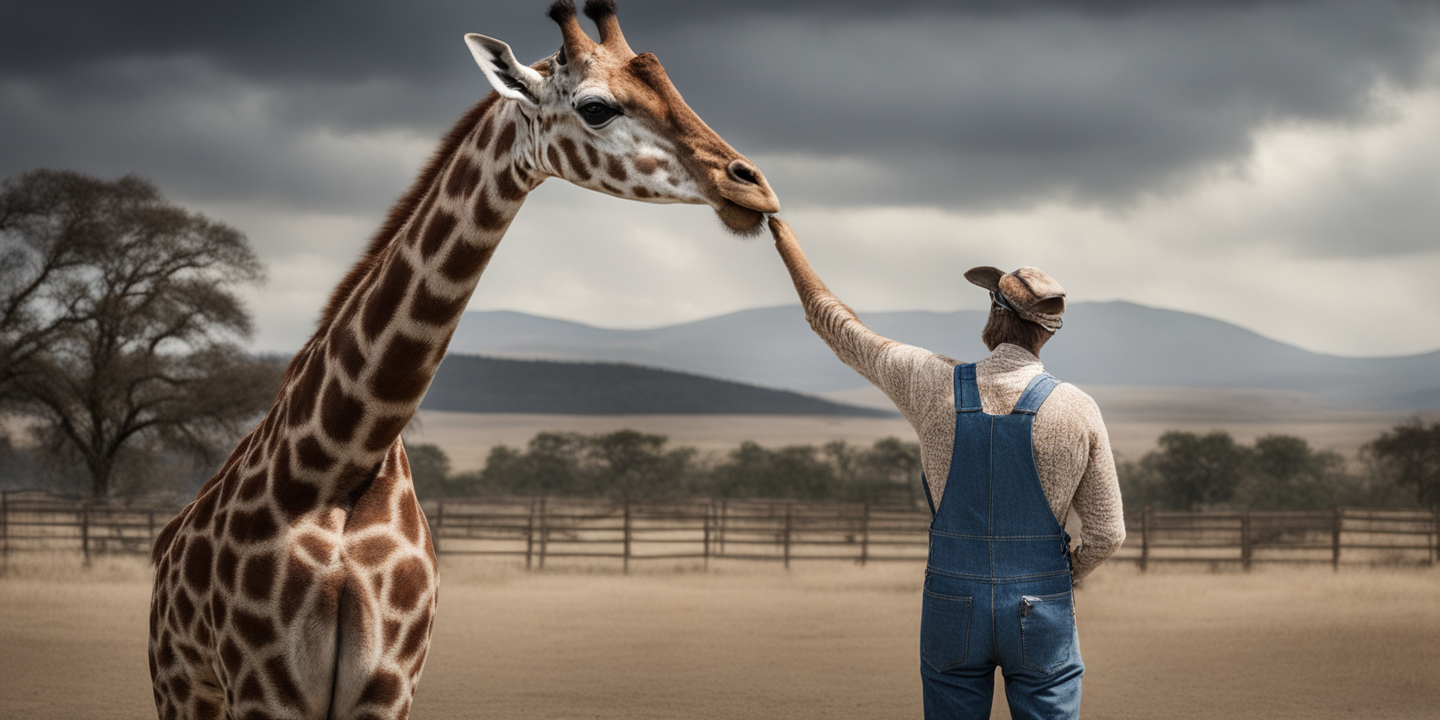
Introducing PatchTST and PatchTSMixer: Enhanced AI Models for Time Series Analysis We're thrilled to announce the integration of PatchTST and PatchTSMixer, cutting-edge AI models tailored for time series forecasting and classification, into the renowned 🤗 Transformers library, made possible through a collaborative effort with IBM. PatchTST stands out for its exceptional performance in long-term forecasting tasks, leveraging MAE self-supervision during pre-training to deliver unparalleled results, particularly on complex multivariate time series datasets. Notably, it offers support for zero-shot transfer learning, enabling seamless adaptation to domain-specific datasets without extensive retraining. Complementing PatchTST, PatchTSMixer introduces a lightweight alternative to traditional Transformer architectures, incorporating multi-layer perceptron (MLP) modules to streamline computations while maintaining high performance. With the addition of PatchTST and PatchTSMixer, the Transformers library now offers users access to a comprehensive suite of 5 advanced time series models. This expansion not only broadens the toolkit available to practitioners but also sets the stage for ongoing enhancements and future benchmarking efforts aimed at further refining the performance and versatility of time series analysis methods. Stay tuned for updates as we continue to push the boundaries of AI-driven time series forecasting and classification