Patch and IBM Unveil Time Series Models
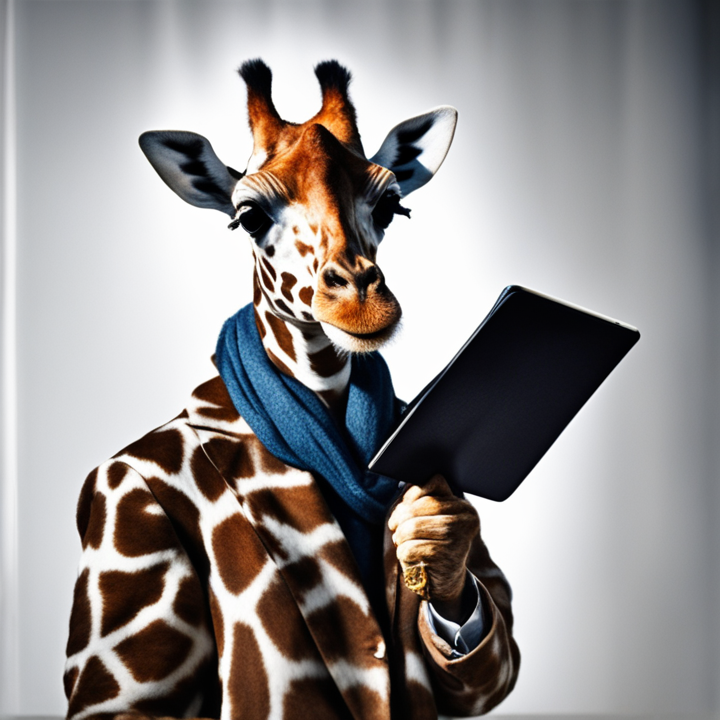
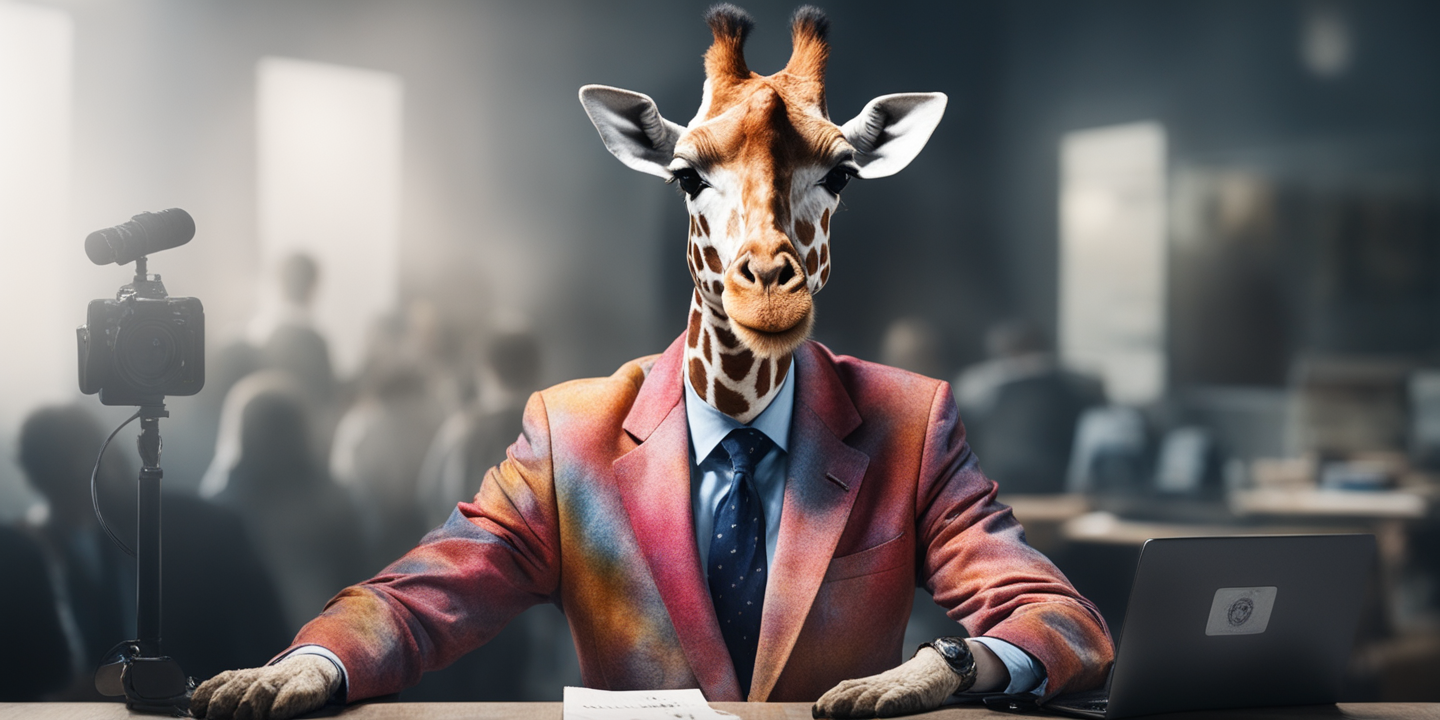
Exciting news from the collaboration between Patch and IBM! Together, they announce the integration of PatchTST and PatchTSMixer into the renowned 🤗 Transformers library, offering powerful AI models tailored for time series forecasting and classification tasks. PatchTST, a standout addition to the library, is designed to handle multivariate time series data with exceptional performance, particularly excelling in long-term forecasting assignments. Utilizing MAE self-supervision during pre-training, PatchTST leverages a unique approach of masking patches of values to reconstruct them based on contextual cues. This innovative technique enables zero-shot transfer learning, allowing seamless adaptation to similar datasets without extensive retraining. On the other hand, PatchTSMixer offers a lightweight alternative within the library, comprising solely of multi-layer perceptron (MLP) modules. This design choice ensures efficient computation, making it an appealing option for scenarios where computational resources are constrained. With the addition of PatchTST and PatchTSMixer, the Transformers library now boasts a total of five dedicated time series models, catering to a diverse range of forecasting and classification needs. Looking ahead, Patch and IBM aim to expand this repertoire further, while also enhancing benchmarking efforts against traditional methodologies. Stay tuned for more advancements as Patch and IBM continue to drive innovation in time series analysis within the AI landscape.