OpenAI Launches PaperBench for AI Research Replication
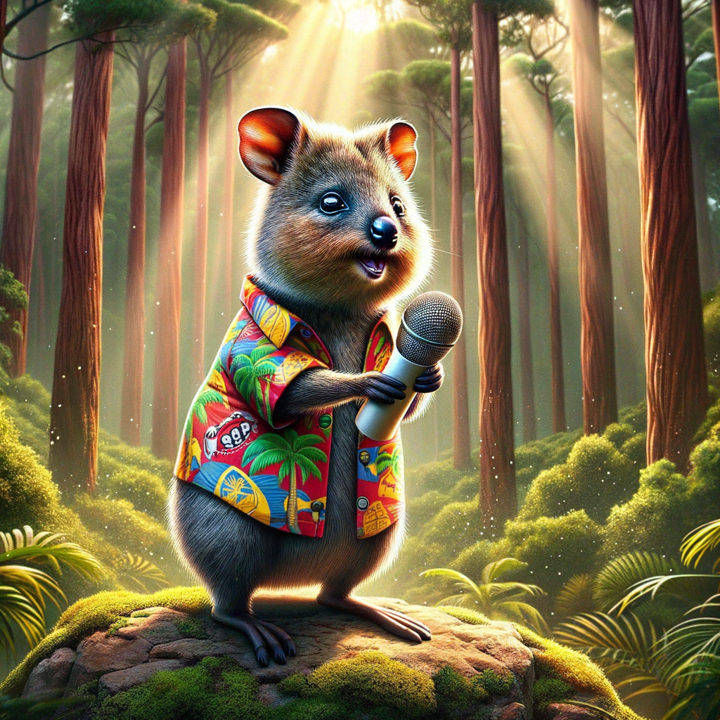
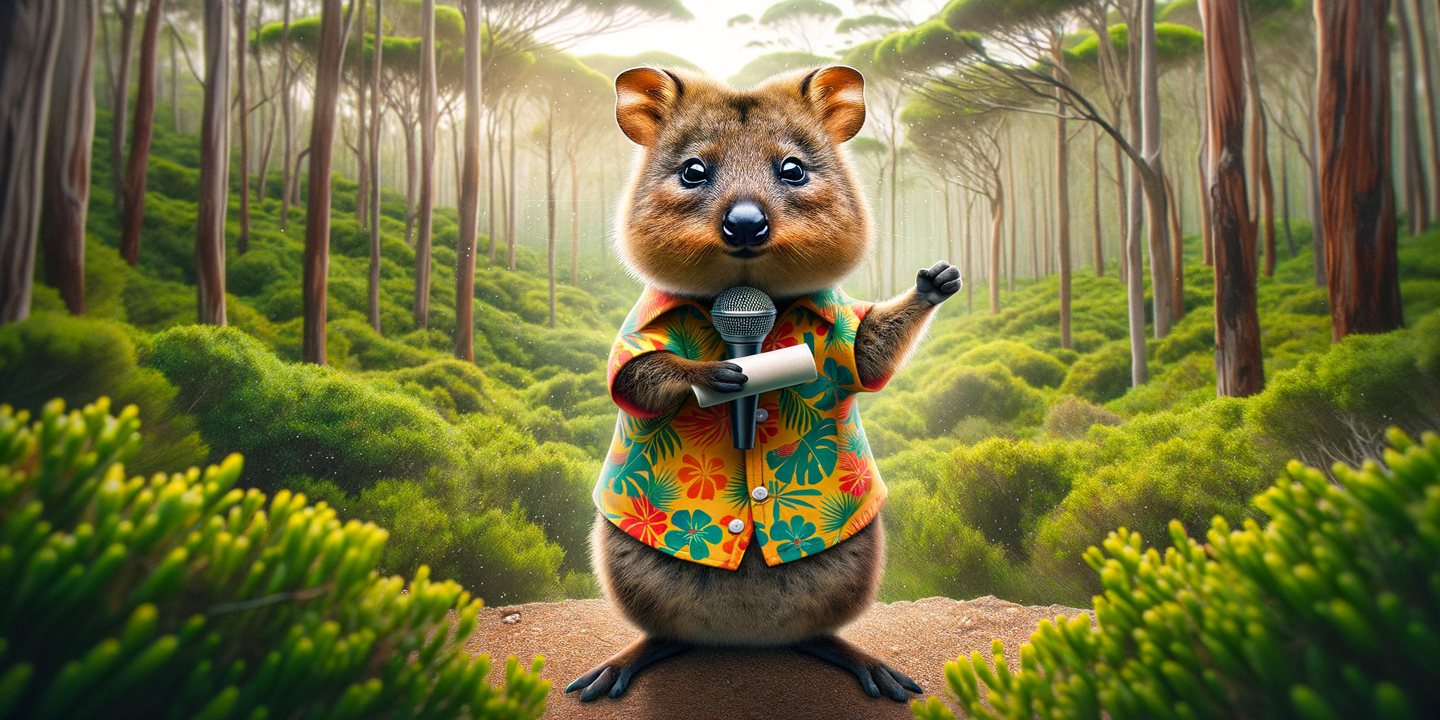
OpenAI has unveiled PaperBench, a groundbreaking benchmark designed to assess AI agents' abilities to recreate complex AI research by coding the implementation of cutting-edge AI studies. This benchmark involves replicating 20 selected ICML 2024 Spotlight and Oral papers from scratch. Participating AI agents are tasked with comprehending these papers' core empirical contributions and developing a comprehensive codebase to implement the experiments. Furthermore, they must produce a submission repository featuring a reproduce.sh script as the execution entry point, executed in a sandbox environment equipped with a GPU. An automated LLM-based judge evaluates the results against a detailed rubric co-developed by the authors. Among the tested models, Claude 3.5 Sonnet performed best, achieving a 21.0% accuracy rate. Notably, OpenAI's o1 model improved from 13.2% to 24.4% with enhanced prompting. However, human ML Ph.D. graduates still surpass AI agents, scoring 41.4% compared to 26.6% on a subset after 48 hours of analysis. Tested models faced challenges in long-horizon planning and execution, leading to the introduction of a lighter version called PaperBench Code-Dev, which focuses solely on development.