MoA Outperforms GPT-4 Omni on Key Benchmarks
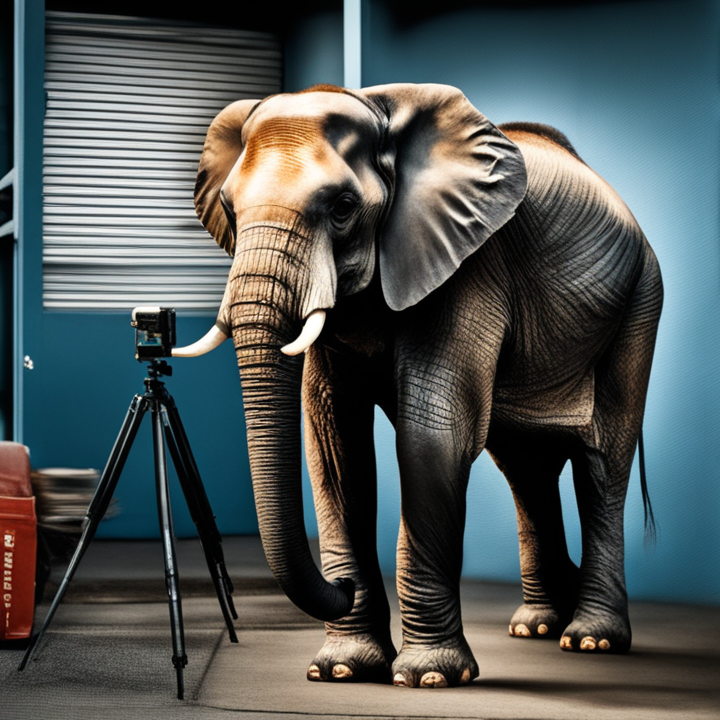
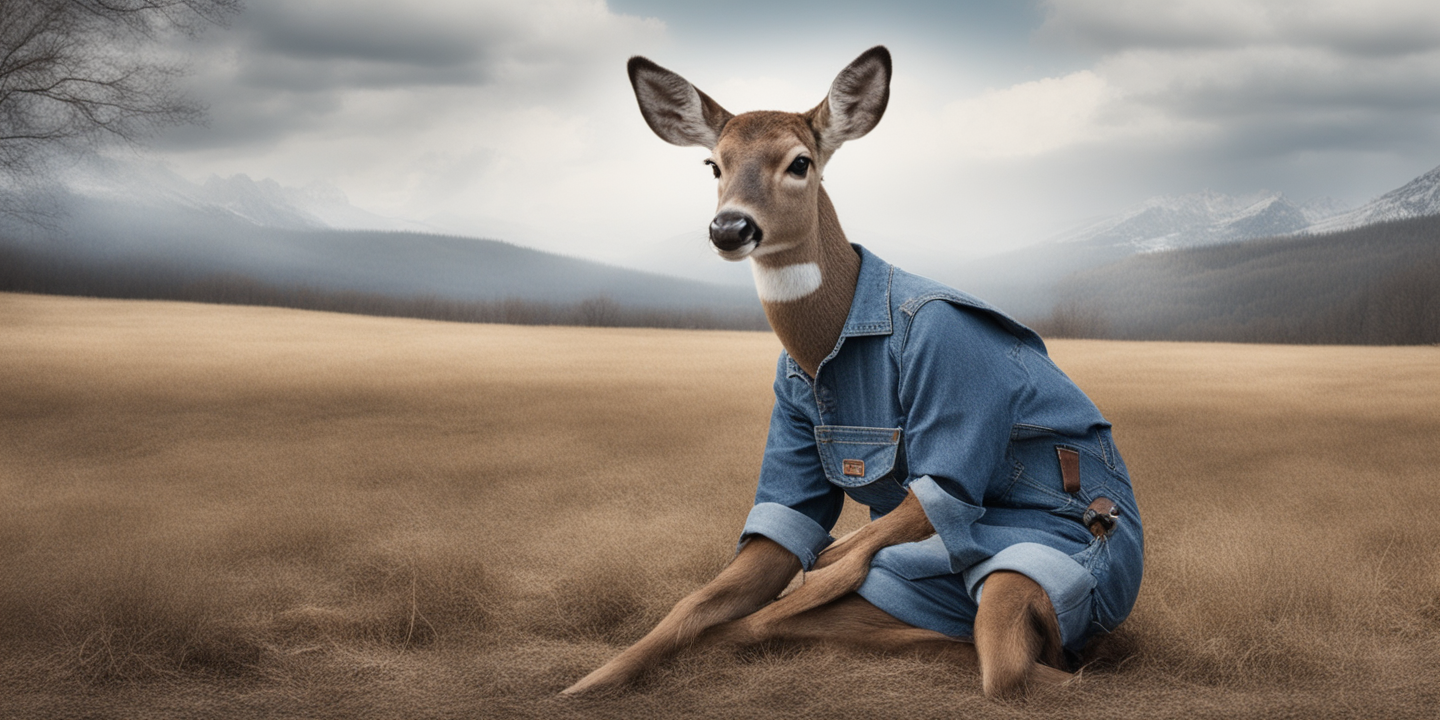
he Mixture-of-Agents (MoA) approach has achieved superior performance over OpenAI's GPT-4 Omni on AlpacaEval 2.0, MT-Bench, and FLASK benchmarks. MoA employs a layered architecture that utilizes multiple Large Language Models (LLMs) to iteratively enhance the quality of generated outputs. This method involves selecting LLMs with diverse strengths and organizing them in layers, where each layer builds on the outputs of the previous one. MoA models scored 65.1% on AlpacaEval 2.0, significantly surpassing GPT-4 Omni's 57.5%. Insights reveal that LLMs generate improved responses when leveraging outputs from other models and that layered aggregation enhances response quality. The MoA-Lite variant even outperforms GPT-4 Turbo by 4% while being twice as cost-efficient. Though effective, this approach can increase Time to First Token (TTFT). MoA shows promise for synthetic data generation and evaluation.