MM1 Insights: Multimodal LLM Pre-training
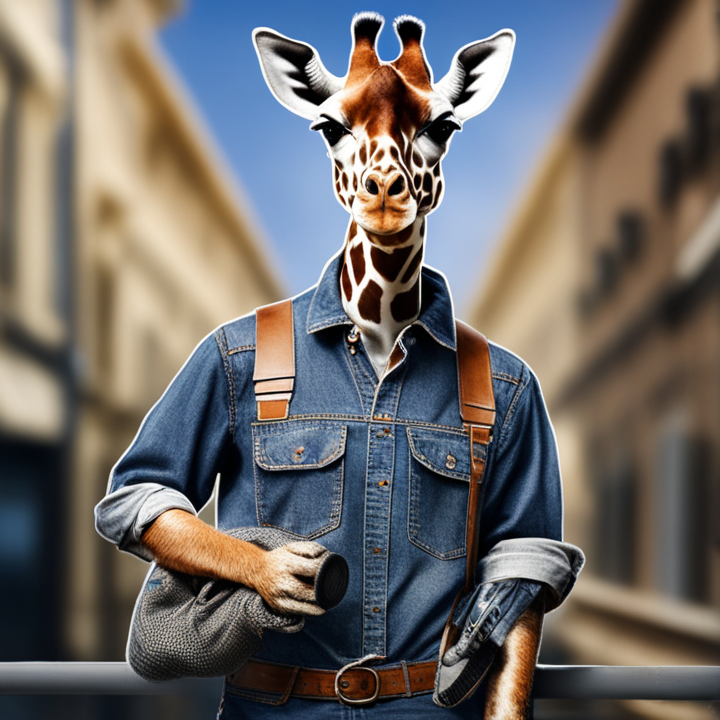
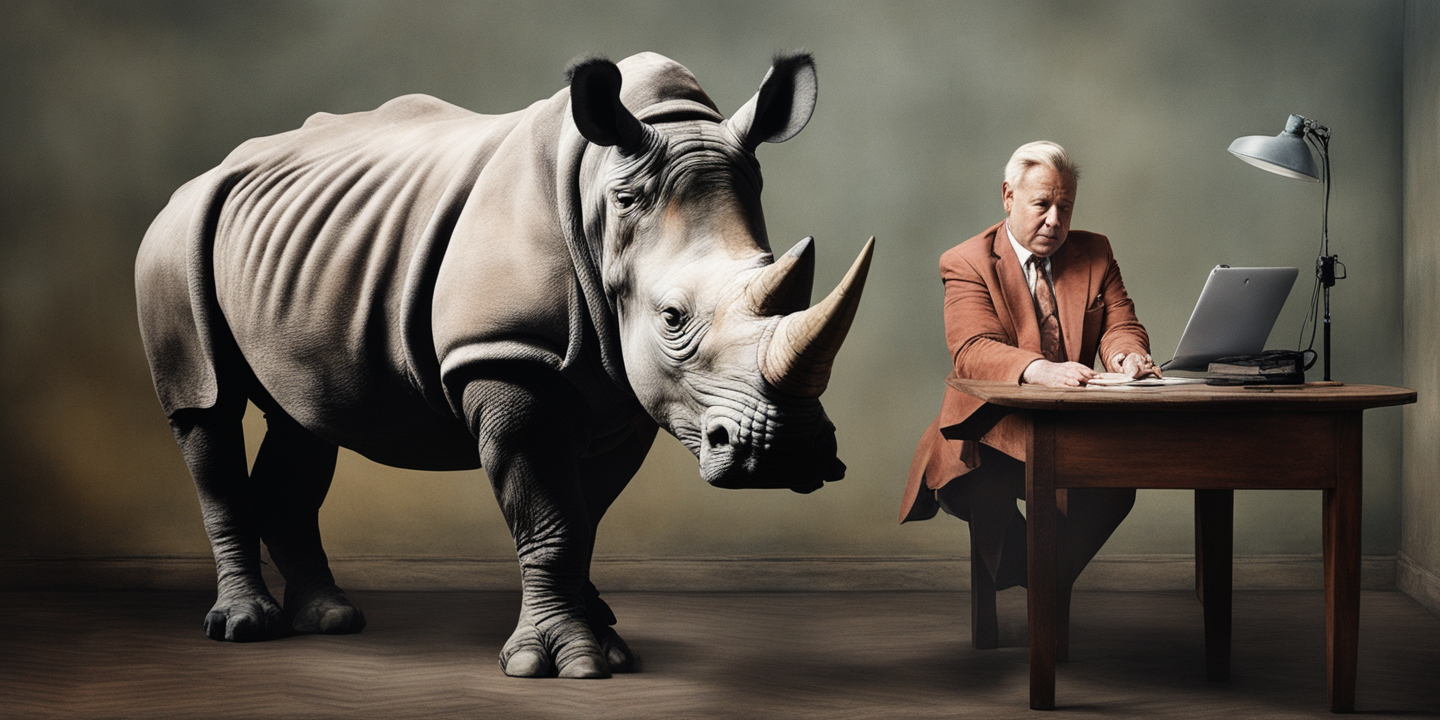
In this comprehensive study, we delve into the intricate process of constructing high-performing Multimodal Large Language Models (MLLMs). Our research meticulously examines the significance of various architectural components and data selection criteria. Through extensive ablation studies involving the image encoder, vision language connector, and pre-training data choices, we unveil pivotal insights into effective model design. Notably, our findings underscore the critical importance of employing a judicious combination of image-caption, interleaved image-text, and text-only data for achieving state-of-the-art (SOTA) few-shot results across diverse benchmarks, surpassing existing pre-training benchmarks. Moreover, our investigation reveals the substantial impact of factors such as image resolution, token count, and the architecture of the image encoder on model performance, while highlighting the relatively lesser importance of the vision-language connector design. Leveraging these insights, we introduce MM1, a versatile family of multimodal models scalable up to 30B parameters. Comprising both dense models and mixture-of-experts (MoE) variants, MM1 not only sets new standards in pre-training metrics but also demonstrates competitive performance upon supervised fine-tuning across various established multimodal benchmarks. Enabled by large-scale pre-training, MM1 exhibits compelling capabilities such as enhanced in-context learning and multi-image reasoning, facilitating few-shot chain-of-thought prompting. Our work represents a significant milestone in the development of MLLMs, offering valuable guidance for constructing performant models capable of tackling diverse multimodal tasks with unparalleled efficacy and versatility.