LLM Creation Insights: AI's YI Family Unveiled
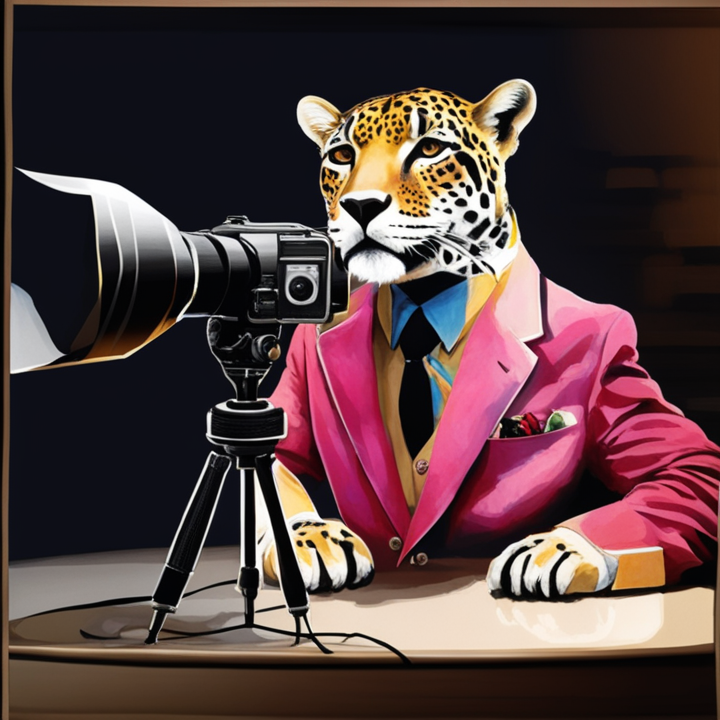
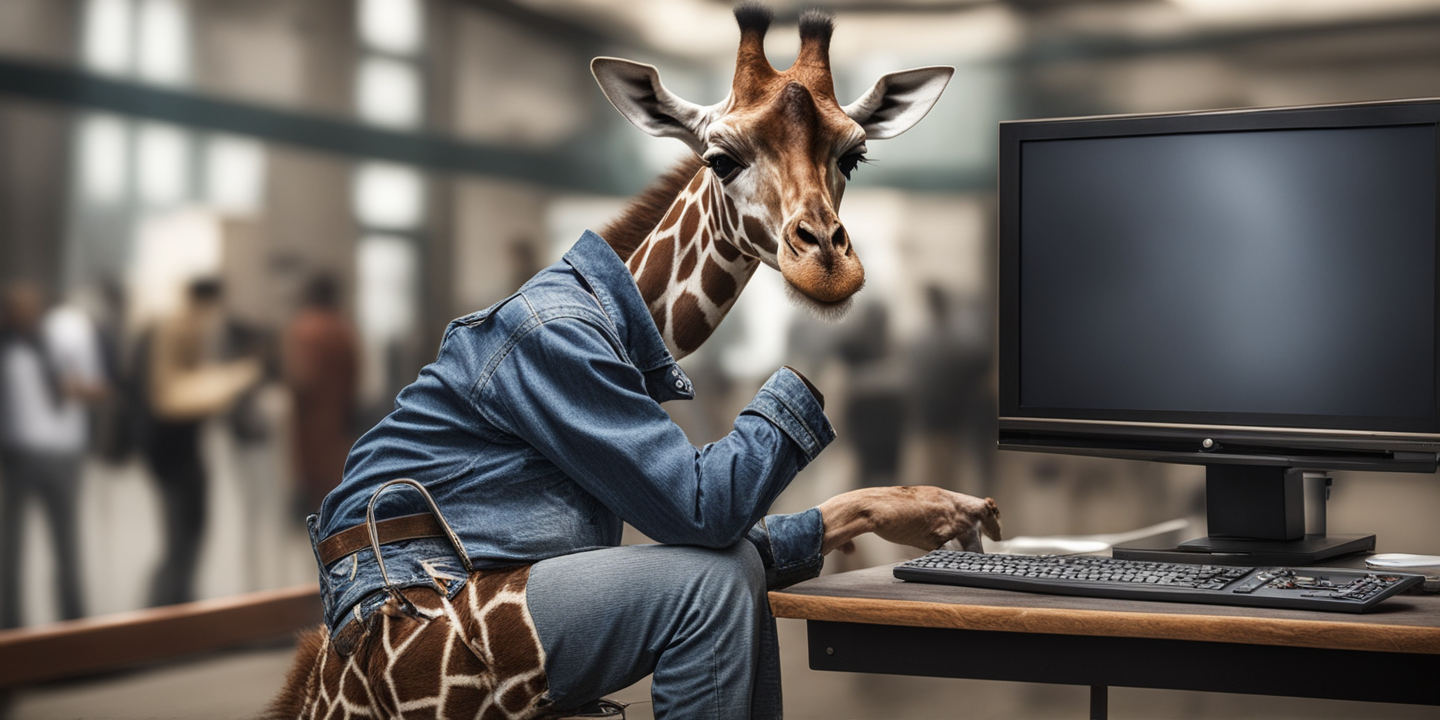
In the landscape of natural language processing, the year 2024 marks a significant milestone in the evolution of open Language Model (LLM) development and training methodologies. Recently, AI has unveiled their comprehensive paper detailing the creation process behind the YI, a groundbreaking family of LLMs and V-LLMs. This paper not only sheds light on the intricacies of data processing but also delves into the nuances of training techniques and the incorporation of multimodality aspects. The journey of crafting the YI family begins with meticulous data processing steps: Data Collection: Leveraging web documents sourced from Common Crawl, AI initiates the process with the assistance of the CCNet pipeline, employing language identification and perplexity scoring mechanisms. Quality Assurance: Rigorous heuristic rules are applied to sift through the data, eliminating low-quality text based on various parameters such as URL, domain, document length, and content coherence. Filtering and Clustering: Advanced filters, including perplexity scorers and quality classifiers, coupled with clustering techniques, aid in the identification and removal of subpar content. Deduplication and Categorization: Further refinement is achieved through deduplication processes at both document and sub-document levels, followed by thematic categorization to streamline the pretraining dataset. Moving beyond data preprocessing, the paper dives into the methodologies employed in model pre-training, fine-tuning, and the integration of multimodality: Pre-training Strategies: Beginning with the Llama 2 architecture, enhancements such as Grouped-Query Attention and SwiGLU activation are incorporated. The model undergoes successive pretraining phases, culminating in refined iterations tailored for specific contexts. Fine-tuning Techniques: Specialized datasets are curated for fine-tuning, particularly in domains like multi-turn instruction-response dialog pairs. Advanced techniques like CoT and Evol-Instruct are employed to enhance model performance. Multimodal Integration: A significant leap is made in multimodal capabilities with the introduction of vision encoders, exemplified in the VLLM architecture. Models are trained on large-scale image-text pairs, culminating in impressive multimodal understanding and performance metrics. Noteworthy among these advancements is the emergence of the Yi-9B model, an upscale iteration achieved through intricate model architecture adjustments and extensive pretraining. This upscale variant exhibits remarkable improvements in key evaluation metrics, showcasing the iterative nature of LLM development and training methodologies. In summation, AI's unveiling of the YI family not only represents a milestone in LLM evolution but also underscores the complexity and ingenuity required in crafting state-of-the-art models equipped to tackle the multifaceted challenges of natural language understanding and multimodal comprehension in the contemporary landscape.