LLaVA-NeXT: Advancing Multimodal Capabilities
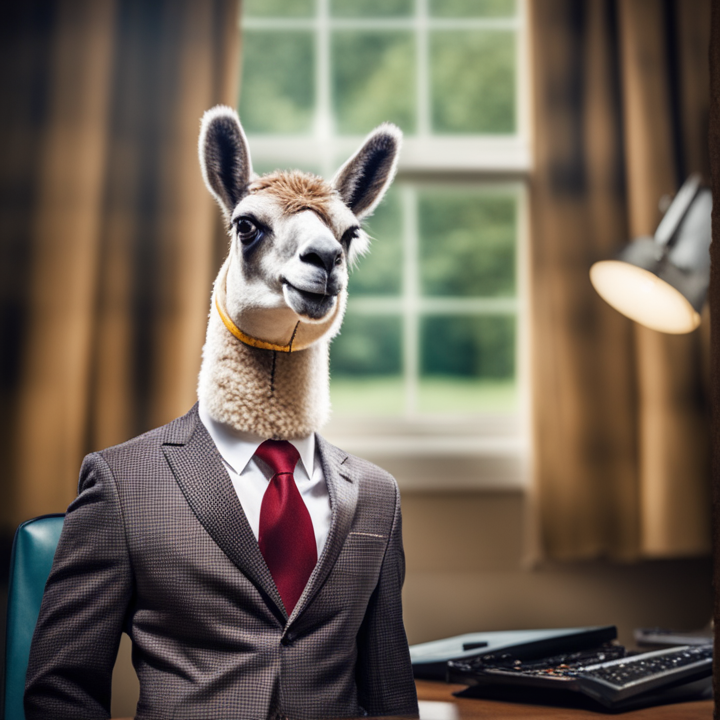
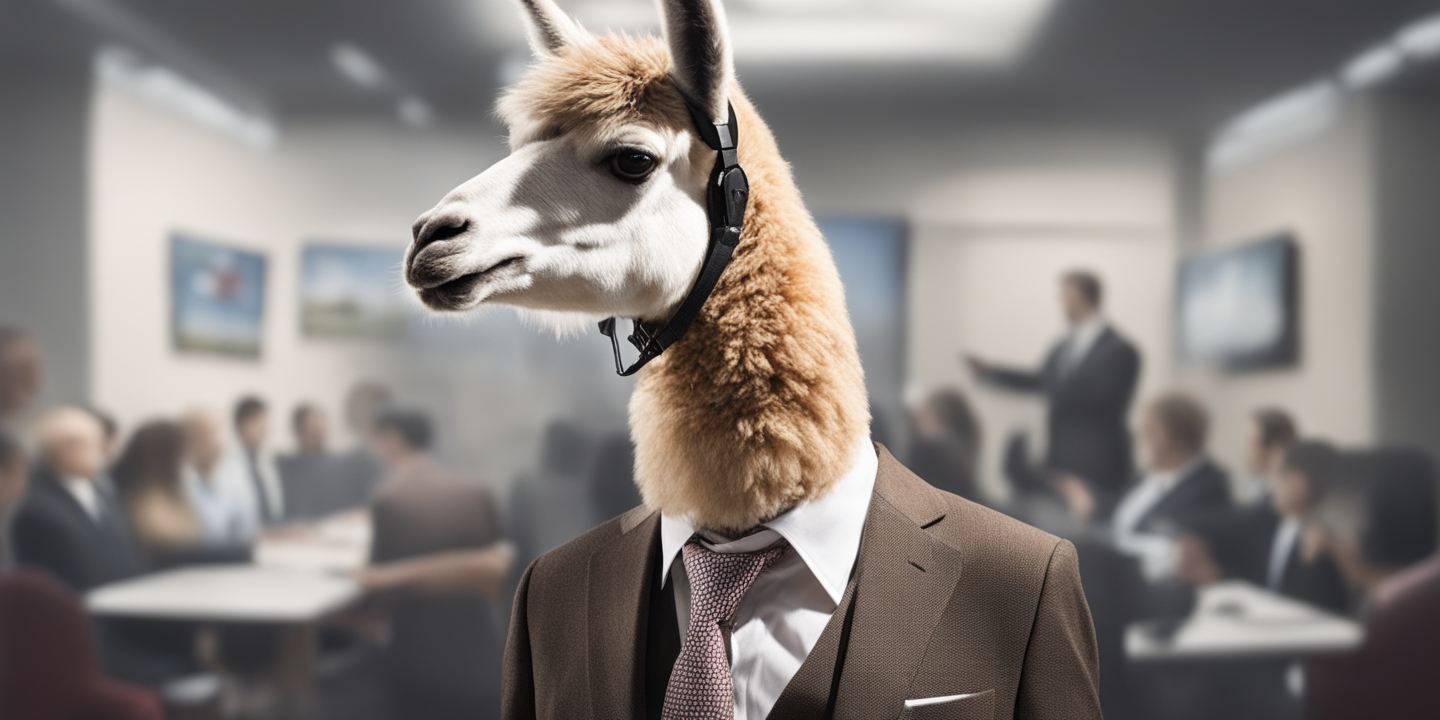
LLaVA-NeXT, introduced in the research paper "LLaVA-NeXT: Improved reasoning, OCR, and world knowledge" by Haotian Liu, Chunyuan Li, Yuheng Li, Bo Li, Yuanhan Zhang, Sheng Shen, and Yong Jae Lee, represents a significant leap forward in multimodal model capabilities. Building upon the success of its predecessor, LLaVA-1.5, which boasted efficient design and exceptional performance across a range of datasets, LLaVA-NeXT raises the bar with enhanced reasoning, Optical Character Recognition (OCR), and world knowledge integration. Notably, LLaVA-NeXT surpasses Gemini Pro on multiple benchmark tests. Key improvements over LLaVA-1.5 include: Increased input image resolution, providing four times more pixels and enabling a deeper understanding of visual details across various aspect ratios. Enhanced visual reasoning and OCR capabilities through a refined visual instruction tuning dataset. Expanded applicability in visual conversation scenarios, catering to diverse applications. Strengthened world knowledge and logical reasoning abilities. Efficient deployment and inference facilitated by SGLang. Despite these advancements, LLaVA-NeXT maintains the minimalist design and data efficiency of its predecessor, utilizing less than 1 million visual instruction tuning samples. Even the largest 34-billion-parameter variant achieves training completion in approximately one day with 32 A100s.