JetMoE: Llama2 Performance at $0.1M
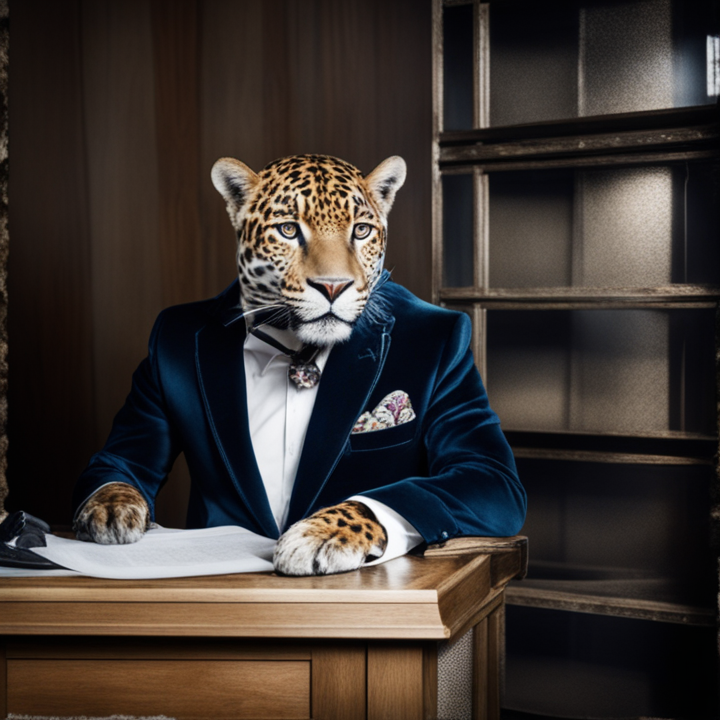
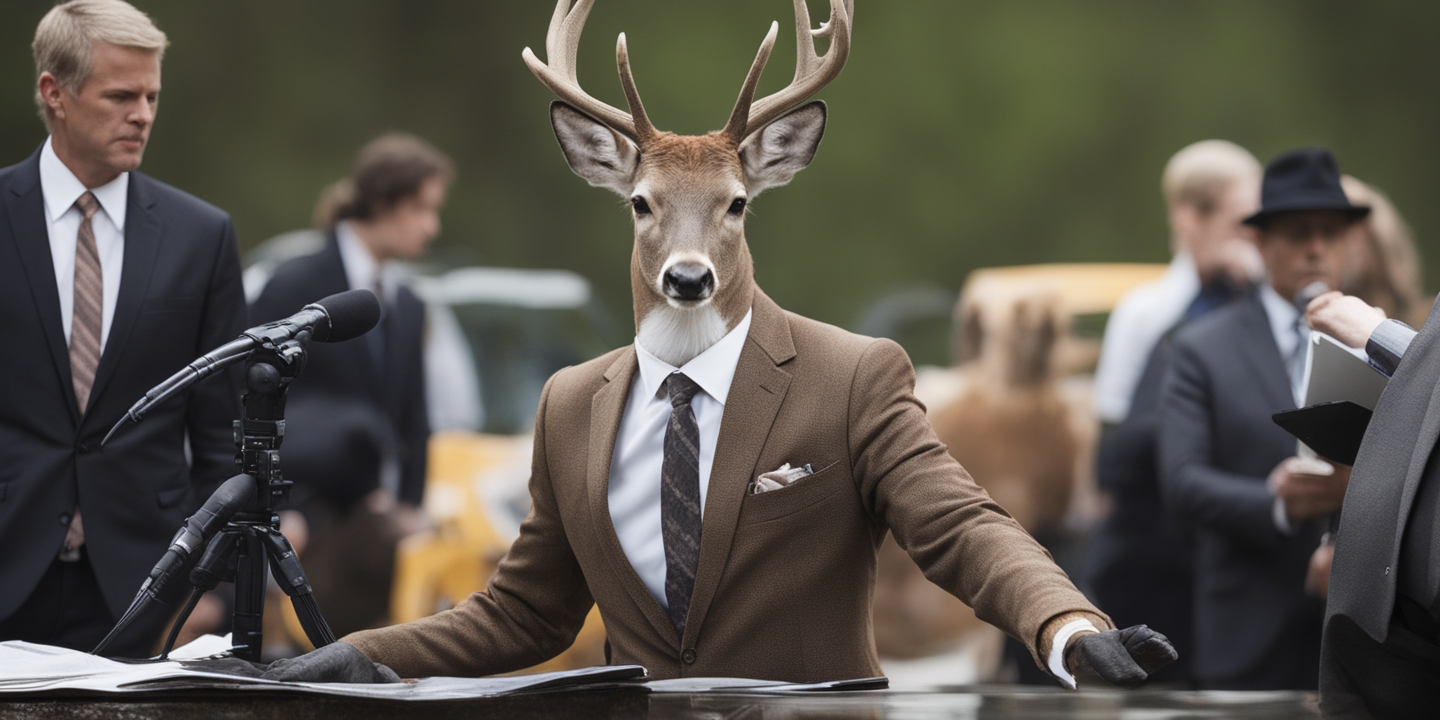
Delve into the groundbreaking research of JetMoE, a new marvel in the realm of natural language processing, boasting Llama2 (7B) performance with an 8B-MoE architecture. Despite a modest $0.1M budget and a token count of just 1.25 trillion, JetMoE achieves remarkable results by employing a novel multi-stage training approach. This approach, akin to Mixtral or DBRX, harnesses a sparsely Gated Mixture-of-Experts (SMoE) architecture, with attention and feedforward layers selectively activated. The journey of JetMoE-8B unfolds across meticulously crafted phases: initialization, dual pretraining stages spanning vast corpora like RefinedWeb and Arxiv, supervised fine-tuning via the Zephyr Recipe, and precision honing through techniques like DPO and Ultrafeedback. Noteworthy insights emerge, highlighting the pivotal role of data quality and the efficacy of Warmup-Stable-Decay (WSD) learning rates in multi-phase pretraining. With a staggering 30,000 H100 GPU hours invested, JetMoE-8B surpasses Llama 2 7B on the Open Leaderboard while achieving a 70% reduction in inference speed through judicious MoE utilization. JetMoE-8B stands as a testament to the power of innovation and resourcefulness in advancing the frontiers of natural language understanding.