Informer Lands on Hugging Face
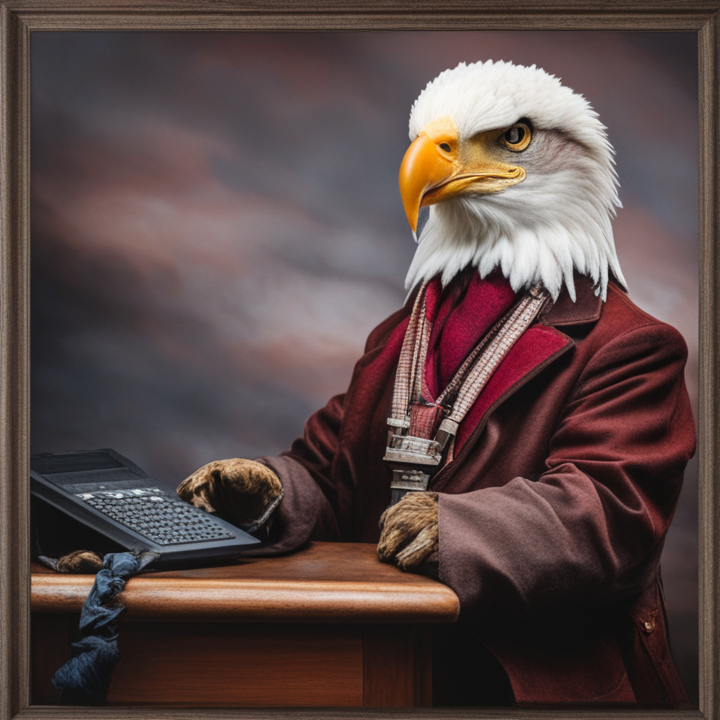
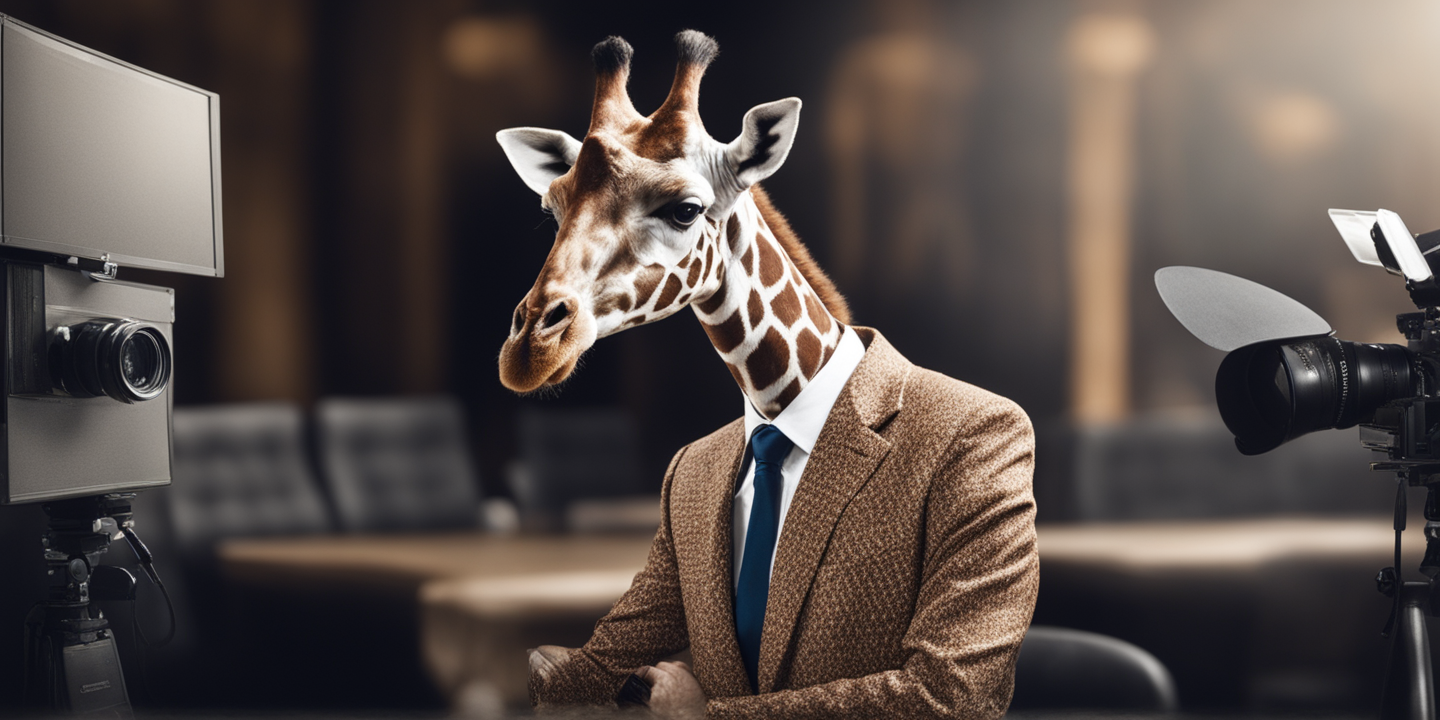
Exciting news for the AI community as the acclaimed Informer model, hailing from the renowned paper "Beyond Efficient Transformer for Long Sequence Time-Series Forecasting" (awarded Best Paper at AAAI'21), is now readily accessible on Hugging Face Transformers. Informer's integration marks a significant leap forward in time-series forecasting capabilities, offering a solution to the challenges posed by long sequence lengths. While the vanilla Transformer, a stalwart in seq2seq modeling, boasts versatility, its quadratic attention scaling in sequence length has hindered its efficacy in long-range forecasting. Informer addresses this hurdle with innovative solutions. Firstly, it introduces a groundbreaking ProbSparse self-attention mechanism, optimizing computational efficiency without compromising performance. Additionally, a distillation operation strategically reduces input size at each layer, ensuring scalability and robustness. To facilitate adoption and exploration, the creators have crafted a comprehensive tutorial for multi-variate forecasting, providing practical insights and guidance for leveraging Informer's capabilities effectively. This resource serves as a valuable asset for practitioners seeking to harness the full potential of Informer in diverse forecasting scenarios. With its arrival on Hugging Face Transformers, Informer promises to empower researchers and practitioners with enhanced tools for tackling complex time-series forecasting tasks, ushering in a new era of efficiency and accuracy in predictive analytics.