Hugging Face's Idefics2 Breakthrough
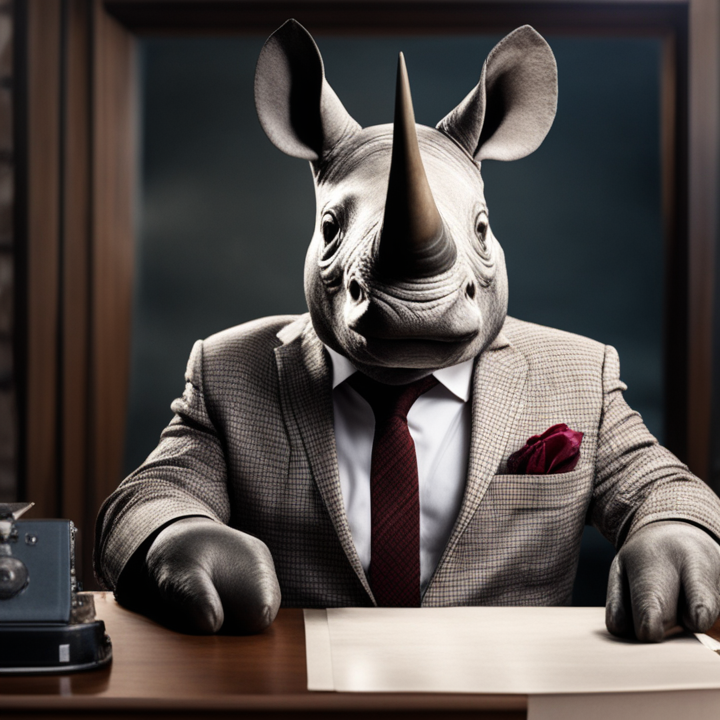
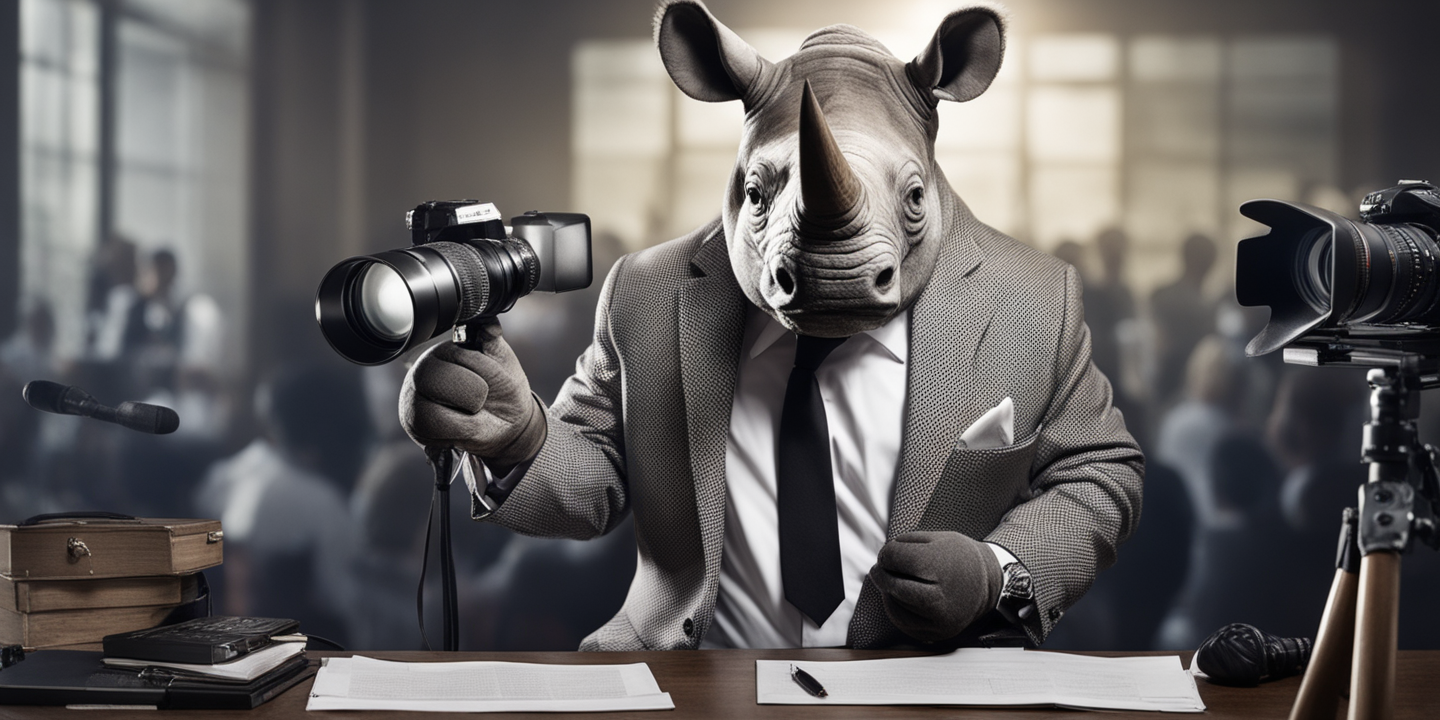
In a significant development for the AI community, Hugging Face has recently introduced Idefics2, its latest open-source vision-language model, marking a substantial leap forward in this burgeoning field. The genesis of Idefics traces back to an attempt to replicate Google DeepMind's Flamingo model, which unfortunately was never made open-source. However, Hugging Face's initial version, accompanied by the Obelics dataset, quickly established itself as a formidable competitor, matching Flamingo's performance across most benchmarks within just a year of release. Now, with the unveiling of Idefics2, Hugging Face has integrated numerous advancements, positioning it as one of the foremost vision-language models, if not the pinnacle, in its class. The team's efforts are documented in a comprehensive paper titled "What matters when building vision-language models?" available at https://lnkd.in/eQdrCYUE. Key enhancements in Idefics2 include the implementation of NaViT patching and Perceiver resampling techniques. NaViT, a novel method developed by Google, intelligently patches images while preserving their aspect ratio, resulting in improved performance, particularly for optical character recognition (OCR) tasks. Meanwhile, Perceiver resampling, an innovation within the Perceiver model, enables encoding vast amounts of visual data into a compact set of latent variables, facilitating efficient representation of complex documents like PDFs with multiple pages. Furthermore, Idefics2 leverages cutting-edge vision and language backbones, building upon SigLIP for the vision encoder and Mistral-7B for the language backbone. This strategic integration significantly enhances its performance across a spectrum of benchmarks, reinforcing its status as a leading contender in the field. To commemorate the release, a demo notebook has been crafted, illustrating how to fine-tune Idefics2 for document AI applications, such as extracting structured data from receipt images, showcasing the model's versatility and potential impact in real-world scenarios.