GLAN: Reshaping LLM Education
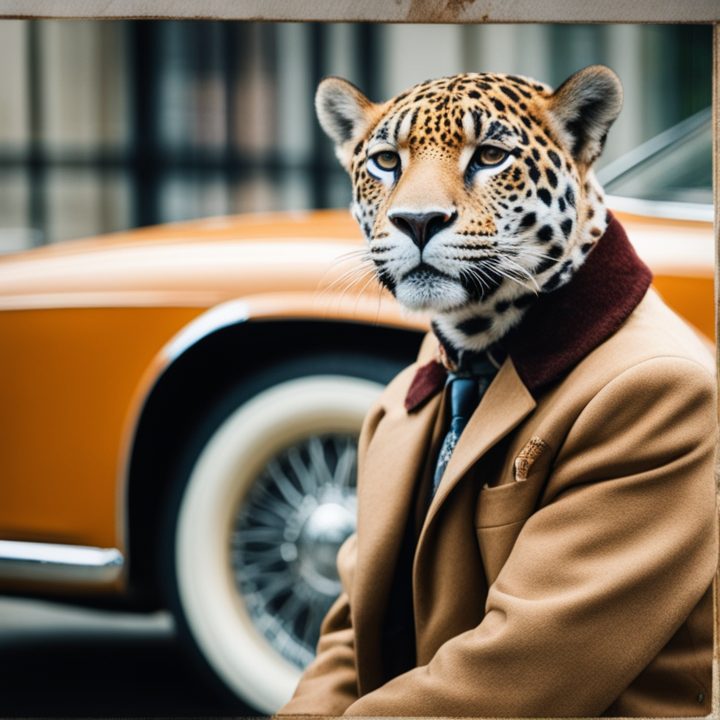
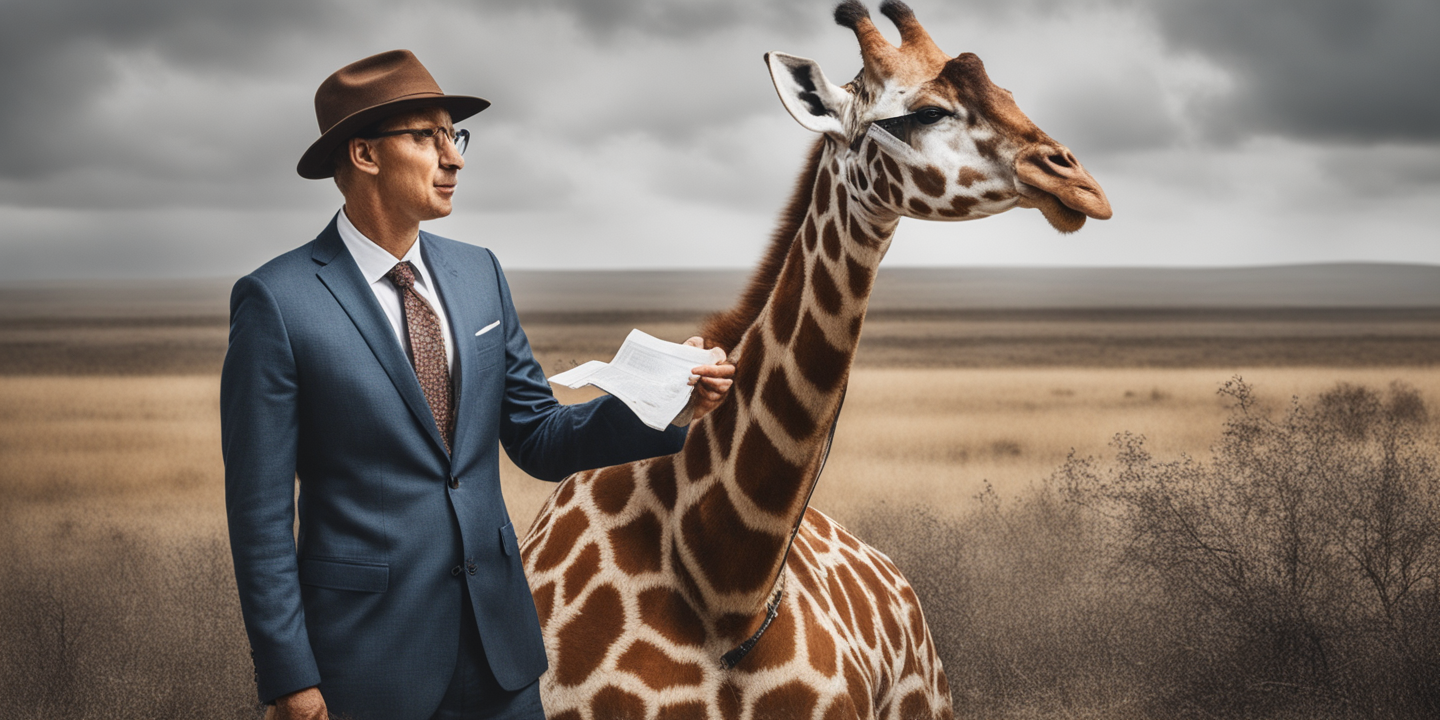
In a groundbreaking development at the intersection of artificial intelligence and education, the question arises: Can Language Learning Models (LLMs) attend school to enhance their learning capabilities? This intriguing proposition is now within the realm of possibility as researchers unveil a pioneering method to train and refine LLMs using synthetic-generated school syllabuses. Enter GLAN, a cutting-edge technique developed by Microsoft, poised to revolutionize the landscape of AI-driven education. Unlike traditional methods that rely on predefined instruction sets, GLAN operates autonomously, leveraging a taxonomy of human knowledge to generate diverse instructional data. By eliminating the need for seed instructions, GLAN promises to deliver unparalleled versatility and depth in LLM training. The implementation of GLAN follows a meticulously structured process: Build a Taxonomy: Utilize human verification to construct a comprehensive taxonomy of human knowledge, encompassing diverse disciplines such as Natural Sciences and Humanities. Generate Subjects: Create an exhaustive list of subjects within each discipline, spanning fields like Computer Science and Sociology. Design Syllabuses: Develop detailed syllabuses for each subject, meticulously breaking down complex concepts into smaller units and class sessions essential for mastery. Generate Instructional Data: Employ synthetic instruction generation techniques to produce a rich array of instructional materials, including homework questions and answers, aligned with the syllabus content. Underpinning each step of the process is the formidable computational power of GPT-4, driving the generation and extraction of data with unparalleled efficiency and accuracy. Leveraging GLAN, powered by the Mistral 7B model, yields significant enhancements across various domains, including mathematics, coding, reasoning, and exam preparation. Moreover, GLAN sets itself apart by ensuring diverse coverage of instructional content, outperforming conventional methods and demonstrating adaptability to domain-specific requirements. However, it's important to note that none of the prompts used in GLAN are released, rendering it challenging to verify the results independently. The introduction of GLAN represents a monumental leap forward in AI-driven education, offering tantalizing prospects for the future of LLM training and proficiency. As researchers continue to push the boundaries of innovation, GLAN stands as a testament to the transformative potential of artificial intelligence in shaping the educational landscape of tomorrow.