Galore: 7B Model Training on Consumer GPUs
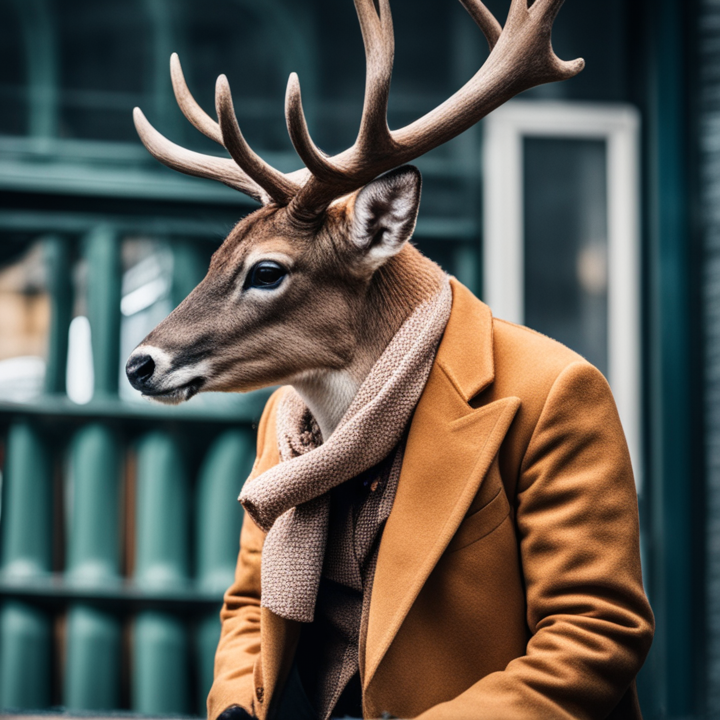
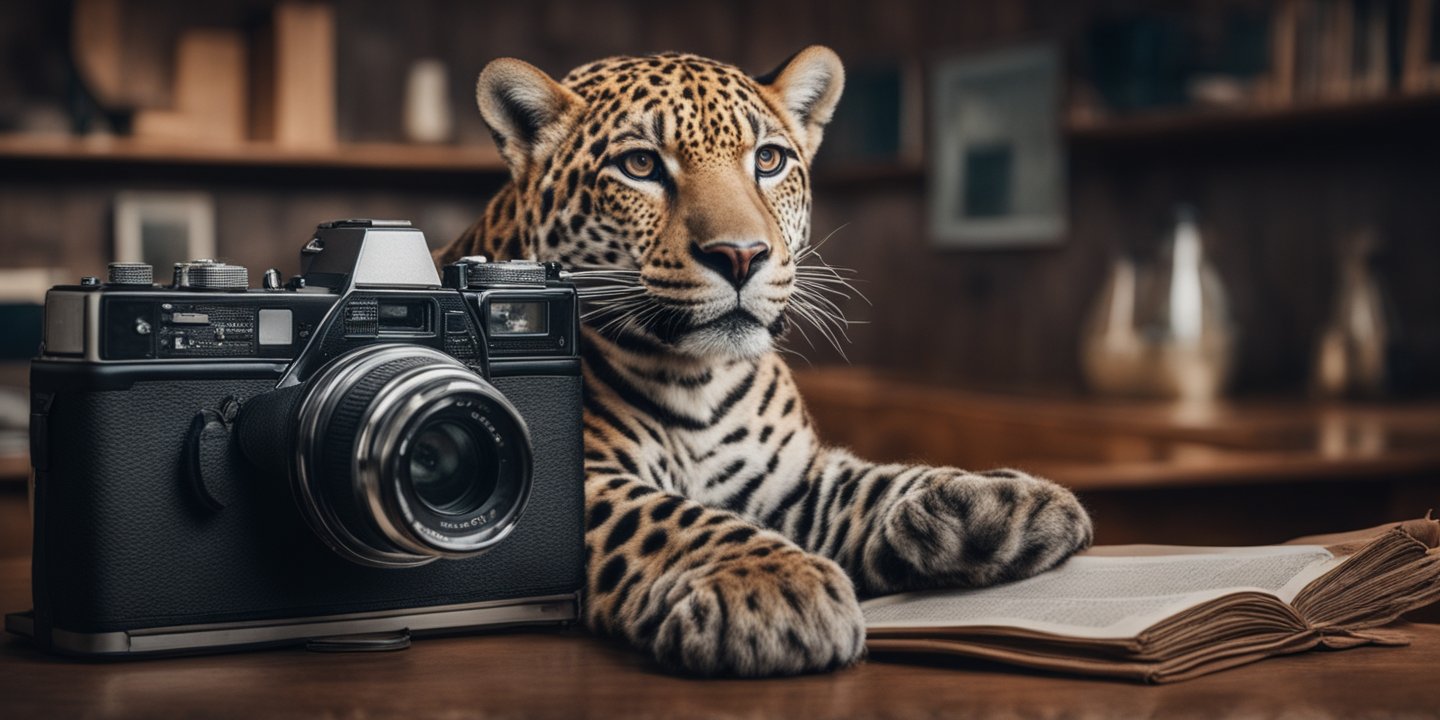
Introducing Galore: Training 7B models on consumer GPUs has never been more accessible and efficient. With this breakthrough, it's now feasible to fully pre-train a 7B model on a consumer-grade GPU boasting 24GB of RAM, all without sacrificing performance! Memory consumption during model training has long posed a significant challenge. Previously, pre-training a 7B model required a whopping ~50GB of RAM. Various strategies have been employed to mitigate this, including distributing models across multiple GPUs (known as "sharding") and quantizing models to reduce memory usage. Another innovative technique involves projecting the weight matrix to a lower-rank space, thereby saving substantial memory. While this method has been explored before, it often resulted in performance degradation, particularly during pre-training. Enter the creators of Galore: Memory-Efficient MLM Training by Gradient Low-Rank Projection. Their research unveils fascinating insights: while the weight matrix may not reliably converge to lower ranks during training, the gradient matrix does. Building on these insights, they introduce Galore, a technique that projects the gradient to lower ranks. By periodically reconstructing the low-rank projection throughout training, they enable optimization to explore a broader space. Moreover, this method can be seamlessly integrated with existing approaches like 8-bit Adam, further enhancing its versatility. The results speak for themselves: Galore achieves a significant reduction in memory footprint, enabling training on consumer-grade GPUs without compromising performance. This scalability extends up to 7B parameters, a validation of its effectiveness and viability.