DPO vs RLHF: Tuning LLMs
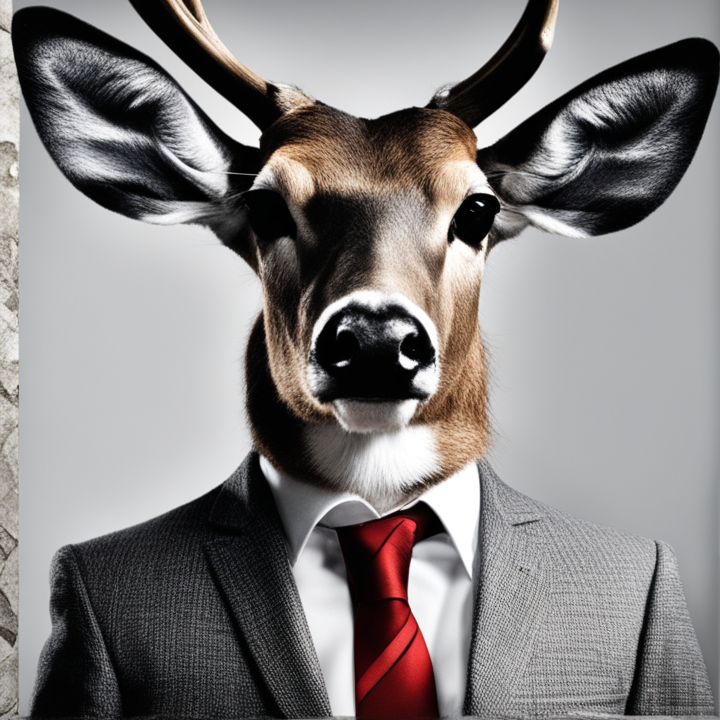
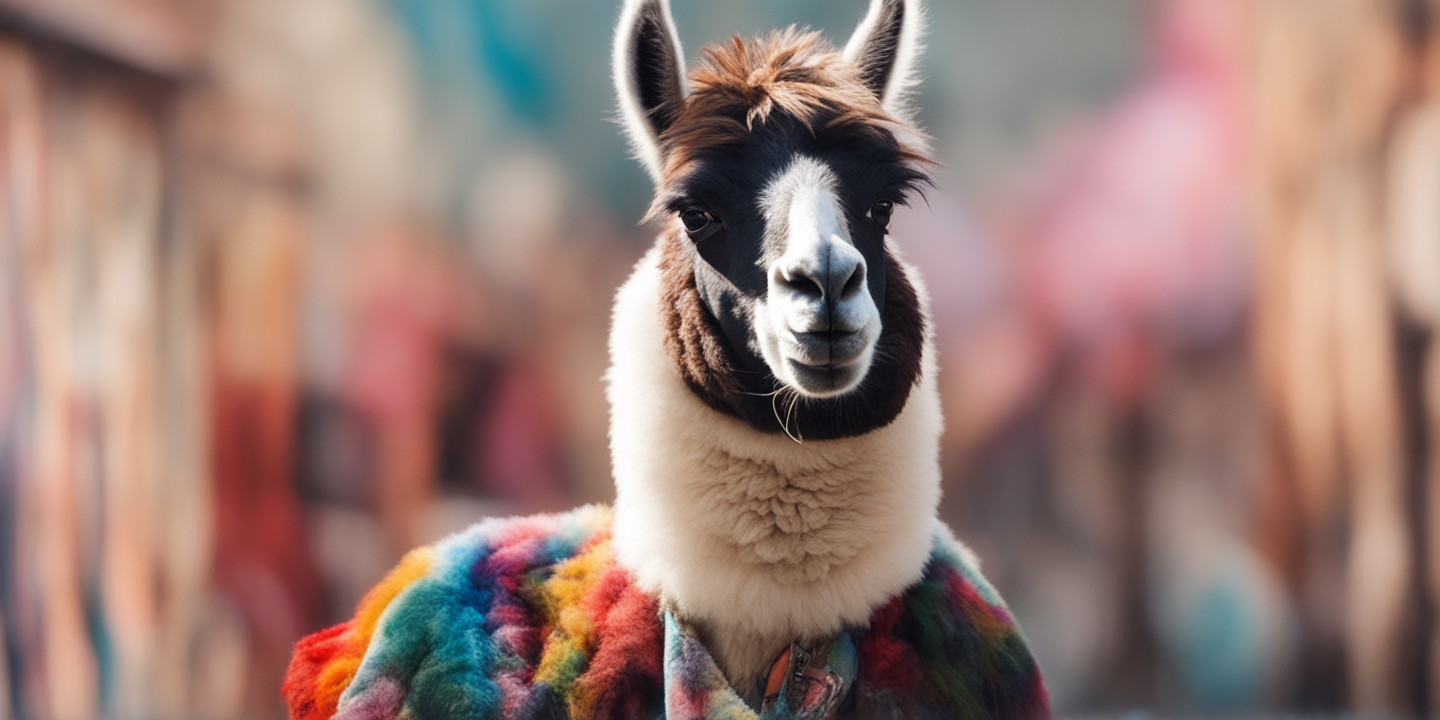
DPO vs RLHF: Tuning LLMs for Optimal Human Interaction" explores the dynamic landscape of preference tuning for large language models (LLMs), juxtaposing the innovative Direct Preference Optimization (DPO) against the established framework of Reinforcement Learning from Human Feedback (RLHF). While RLHF has long been regarded as the cornerstone for aligning AI outputs with human expectations, DPO introduces a streamlined alternative that directly incorporates human feedback. This comprehensive analysis dissects the nuances of both methodologies, shedding light on their respective strengths and limitations. By delving deep into the intricacies of DPO and RLHF, this exploration aims to unveil the most effective pathway for refining LLMs to seamlessly integrate with human interaction, paving the way for transformative advancements in AI technology.