DPO vs PPO: Preference Optimization
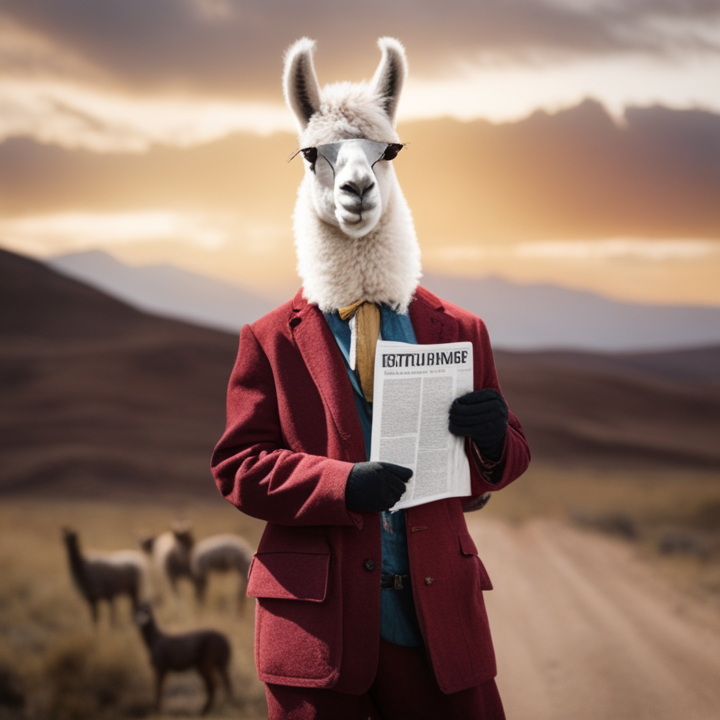
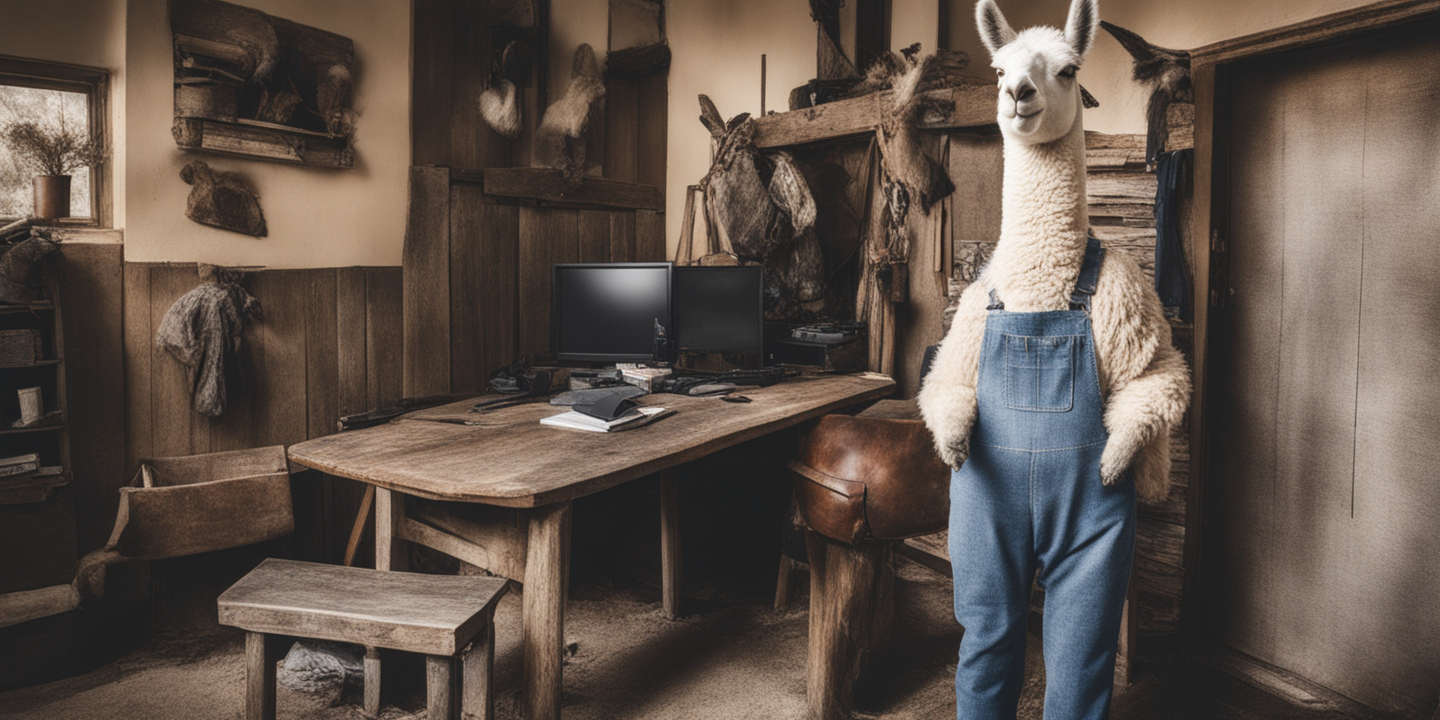
In the realm of optimizing human-derived preferences in language models, traditional methods often rely on reinforcement learning (RL) coupled with auxiliary reward models. These models fine-tune the primary model to maximize a given reward, fostering high-reward outputs while maintaining diversity. However, Direct Preference Optimization (DPO) presents a paradigm shift by sidestepping the reward modeling process altogether. Instead, DPO directly optimizes language models based on preference data. By leveraging an analytical mapping from the reward function to the optimal RL policy, DPO transforms the RL loss into a loss directly over the reference model. This innovative approach not only streamlines the optimization process but also ensures alignment with user preferences from the outset, offering a fresh perspective on preference optimization in language models.