DPO Trainer: Mastering Language Models
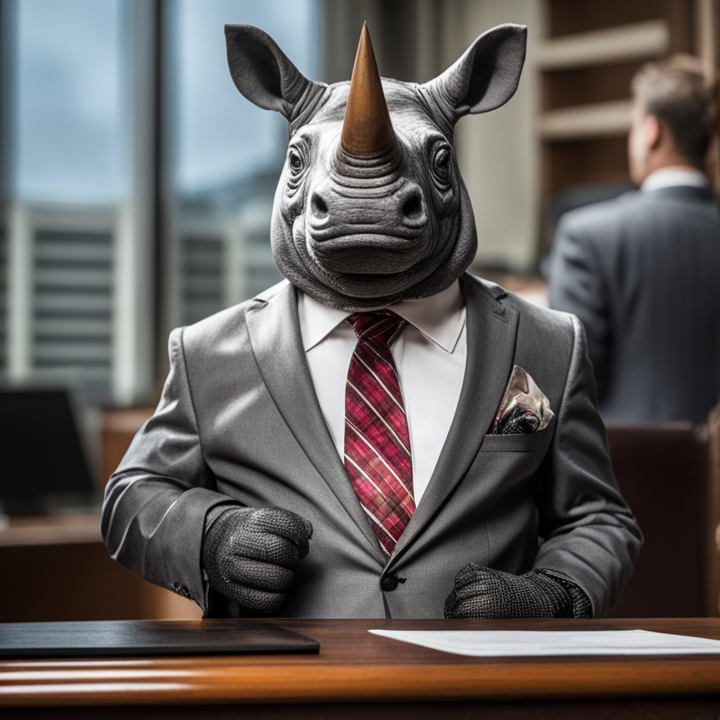
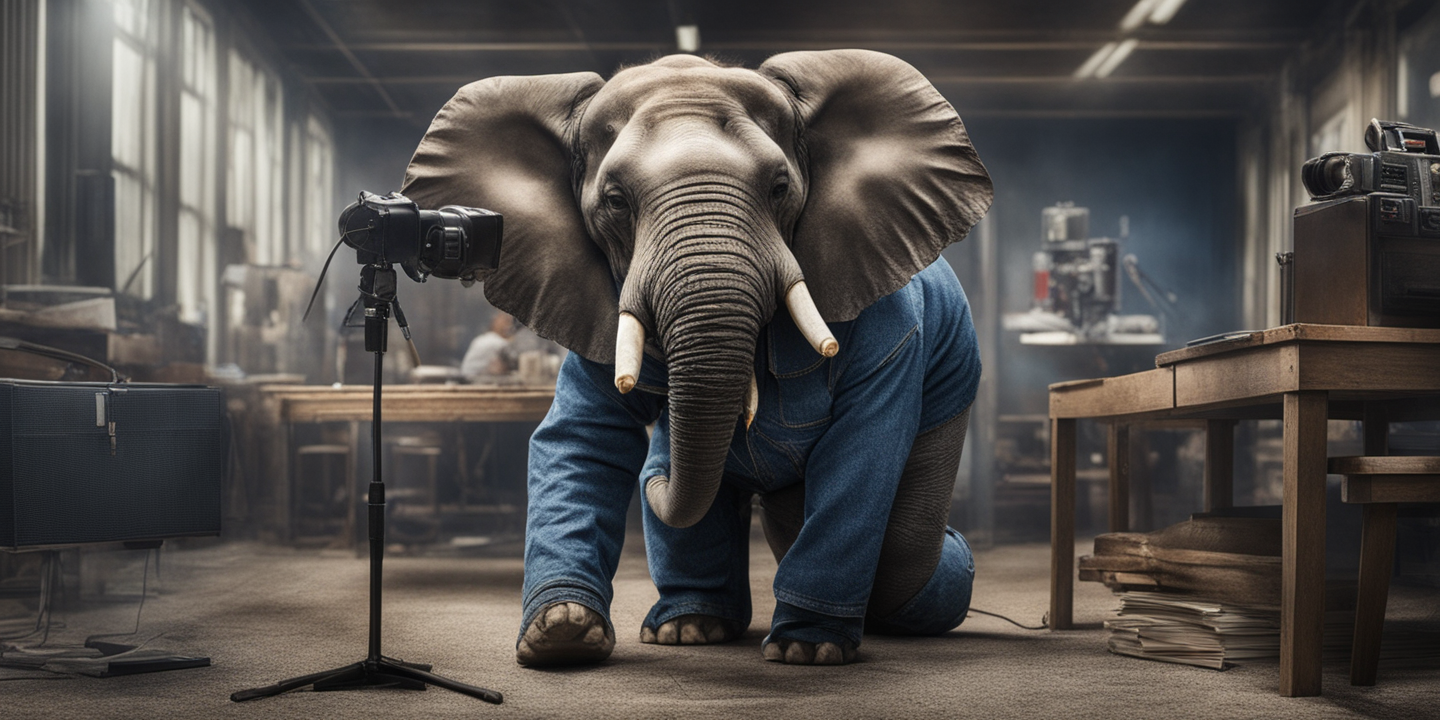
Unlock the power of precise control over language models (LMs) with the groundbreaking Direct Preference Optimization (DPO) method. Developed by Rafael Rafailov, Archit Sharma, Eric Mitchell, Stefano Ermon, Christopher D. Manning, and Chelsea Finn, this innovative approach addresses the challenge of steering the behavior of large-scale unsupervised LMs. While unsupervised LMs excel in learning broad world knowledge and reasoning skills, achieving precise control has proven elusive due to the nature of their training. Existing methods rely on human feedback through reinforcement learning from human feedback (RLHF), but these approaches are complex and often unstable. DPO introduces a new parameterization of the reward model in RLHF, enabling the extraction of the optimal policy in closed form. This novel algorithm simplifies the fine-tuning process, eliminating the need for LM sampling during fine-tuning or extensive hyperparameter tuning. Experimental results demonstrate that DPO can fine-tune LMs to align with human preferences as effectively as or better than existing methods. Notably, DPO outperforms PPO-based RLHF in sentiment control and matches or improves response quality in summarization and single-turn dialogue tasks, all while being substantially simpler to implement and train. Experience the future of LM fine-tuning with DPO Trainer.