Inside DeepSeek v2: Latest Breakthroughs
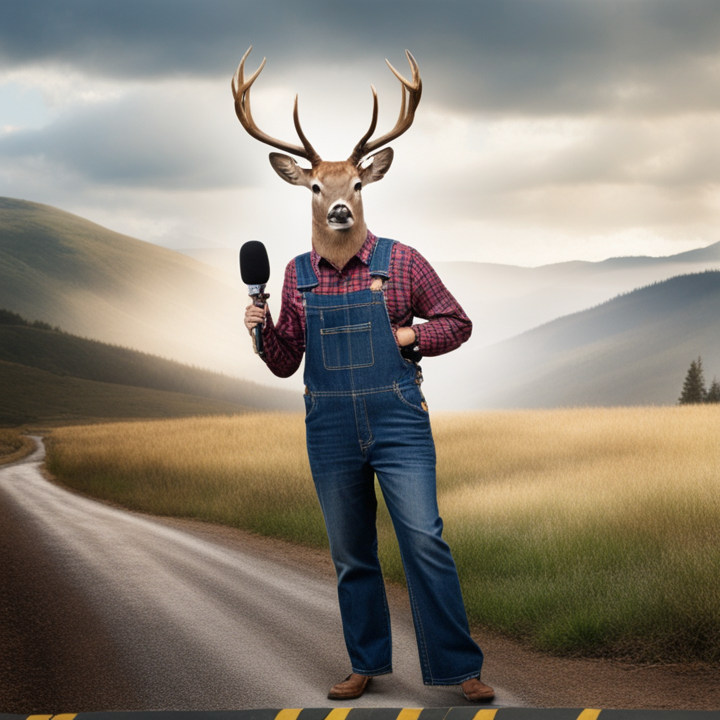
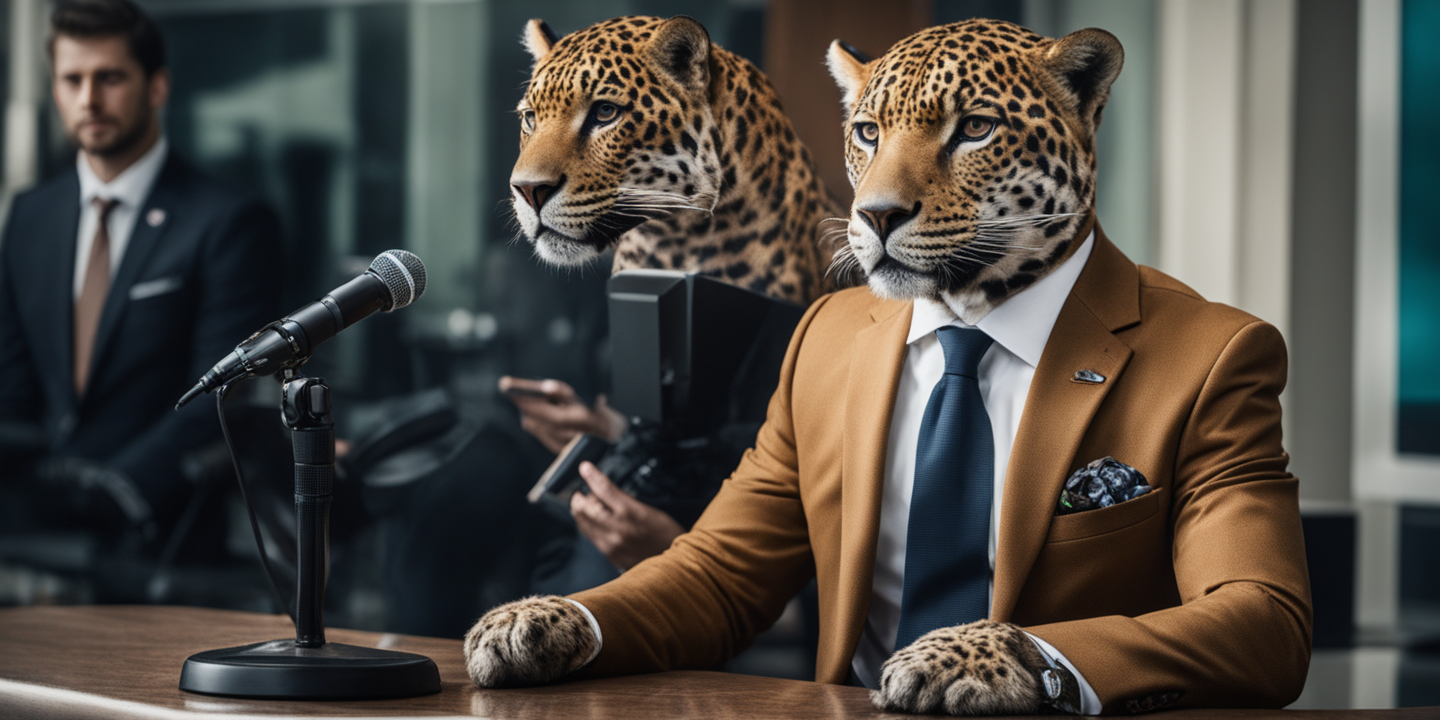
Last week witnessed the release of DeepSeek v2, a monumental achievement in the realm of AI with its 236 billion parameter Mixture-of-Experts (MoE) architecture boasting a 128k context window and 21 billion active parameters. Now, the DeepSeek v2 paper is available, offering a glimpse into its remarkable innovations. The paper unveils a Decoder with Multi-head Latent Attention (MLA) and MoE, where MLA optimizes key-value cache demands by compressing them into a latent vector during inference. Delving deeper, DeepSeek v2 was pretrained on a staggering 8.1 trillion tokens, predominantly English and Chinese, with a sequence length of 4096, followed by Supervised Fine Tuning (SFT) on 1.5 million samples focusing on helpfulness and safety. Notably, Group Relative Policy Optimization (GRPO) aligns model outputs with human preferences, emphasizing instruction following. The learning strategy incorporates nuanced steps, including linear learning rate adjustments and batch size scheduling. Leveraging techniques like YaRN for context window expansion and sparse activation for reduced training costs, DeepSeek v2 demonstrates exceptional performance across metrics like MMLU, AlpacaEval 2.0, and MT-Bench. With 160 experts and hybrid engine deployment, DeepSeek v2 sets a new standard in AI research, now available on Hugging Face for exploration and implementation.