Boosting Financial RAG with Fine-Tuned Embedding Models
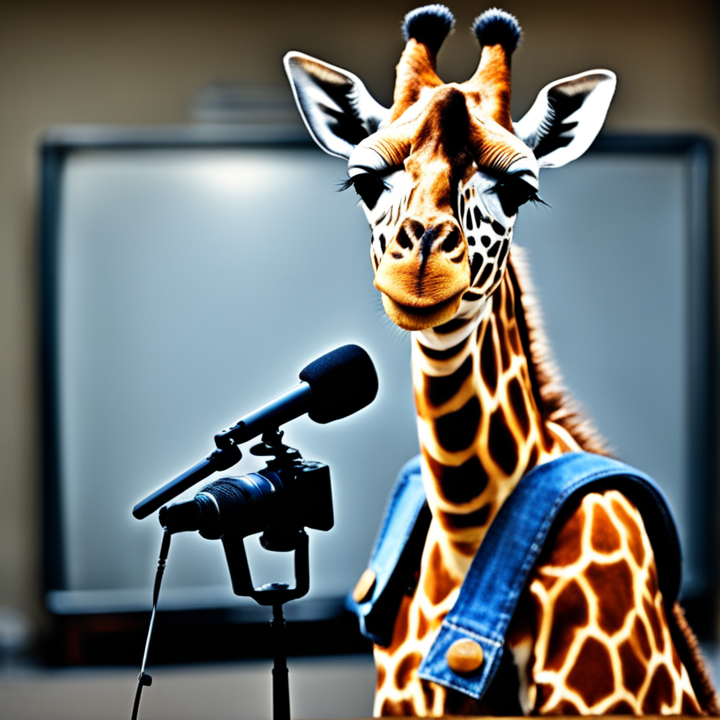
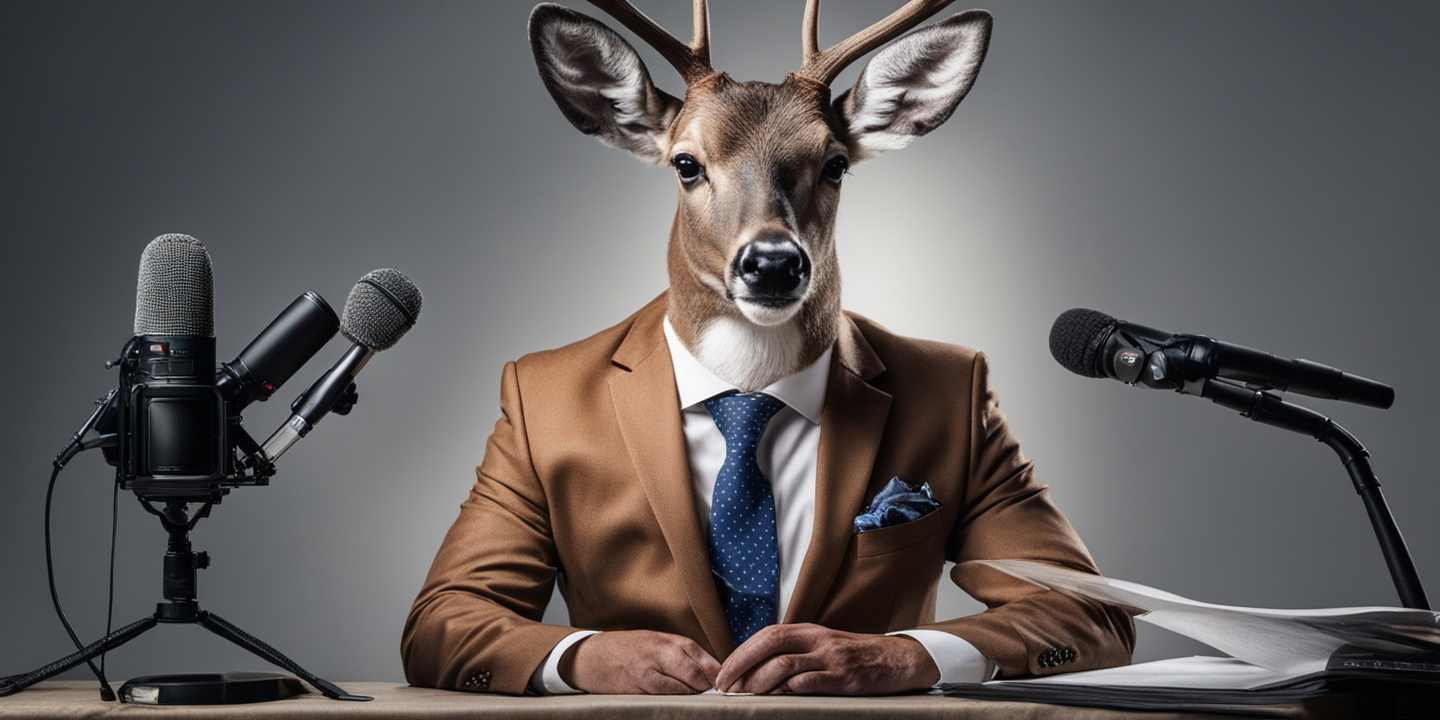
Embedding models are crucial for Retrieval-Augmented Generation (RAG) applications, but general models often fall short in domain-specific tasks. Fine-tuning can significantly enhance retrieval performance. Our latest blog explores how to fine-tune embedding models for financial RAG applications using NVIDIA's 2023 SEC Filing dataset and cutting-edge research, like Matryoshka Representation Learning. Discover how fine-tuning can boost performance by 7.4% to 22.55% with just 6.3k samples. Learn about baseline creation and evaluation during training, and the use of synthetic data for fine-tuning. See how training on approximately 10,000 samples takes only 5 minutes on consumer-grade GPUs. Find out how Matryoshka keeps 99% performance at 6x smaller size, and how a fine-tuned 128-dim model outperforms the baseline 768-dim model by 6.51%. Additionally, this blog utilizes the new Sentence Transformers v3. Dive into the details and elevate your financial RAG applications!